Latest Releases
April 16, 2025
Seedream 3.0 Officially Released
It supports native 2K resolution output, offers faster response speeds, generates more accurate small text, improves text layout effects, enhances aesthetics and structural quality, and demonstrates excellent fidelity and detail performance. It has achieved leading rankings in multiple evaluations.
April 16, 2025
Seedream 3.0 Officially Released
It supports native 2K resolution output, offers faster response speeds, generates more accurate small text, improves text layout effects, enhances aesthetics and structural quality, and demonstrates excellent fidelity and detail performance. It has achieved leading rankings in multiple evaluations.
March 11, 2025
Seedream 2.0 Tech Report
It aims to address critical limitations in existing image generation systems, including model bias, insufficient text rendering capabilities, and deficiencies in understanding culturally nuanced prompts.
March 11, 2025
Seedream 2.0 Tech Report
It aims to address critical limitations in existing image generation systems, including model bias, insufficient text rendering capabilities, and deficiencies in understanding culturally nuanced prompts.
January 22, 2025
Doubao-1.5-pro
It uses MoE , and can surpass the performance of first-class extremely large dense pre-trained models with only a small activation parameter, and achieves excellent results on multiple evaluation benchmarks.
January 22, 2025
Doubao-1.5-pro
It uses MoE , and can surpass the performance of first-class extremely large dense pre-trained models with only a small activation parameter, and achieves excellent results on multiple evaluation benchmarks.
Latest Releases
April 16, 2025
Seedream 3.0 Officially Released
It supports native 2K resolution output, offers faster response speeds, generates more accurate small text, improves text layout effects, enhances aesthetics and structural quality, and demonstrates excellent fidelity and detail performance. It has achieved leading rankings in multiple evaluations.
April 16, 2025
Seedream 3.0 Officially Released
It supports native 2K resolution output, offers faster response speeds, generates more accurate small text, improves text layout effects, enhances aesthetics and structural quality, and demonstrates excellent fidelity and detail performance. It has achieved leading rankings in multiple evaluations.
March 11, 2025
Seedream 2.0 Tech Report
A Native Chinese-English Bilingual Image Generation Foundation Model
It aims to address critical limitations in existing image generation systems, including model bias, insufficient text rendering capabilities, and deficiencies in understanding culturally nuanced prompts.
March 11, 2025
Seedream 2.0 Tech Report
A Native Chinese-English Bilingual Image Generation Foundation Model
It aims to address critical limitations in existing image generation systems, including model bias, insufficient text rendering capabilities, and deficiencies in understanding culturally nuanced prompts.
January 22, 2025
Doubao-1.5-pro
Balance between Superior Model Performance and Optimal Inference Efficiency
It uses MoE , and can surpass the performance of first-class extremely large dense pre-trained models with only a small activation parameter, and achieves excellent results on multiple evaluation benchmarks.
January 22, 2025
Doubao-1.5-pro
Balance between Superior Model Performance and Optimal Inference Efficiency
It uses MoE , and can surpass the performance of first-class extremely large dense pre-trained models with only a small activation parameter, and achieves excellent results on multiple evaluation benchmarks.
View more
Selected Papers
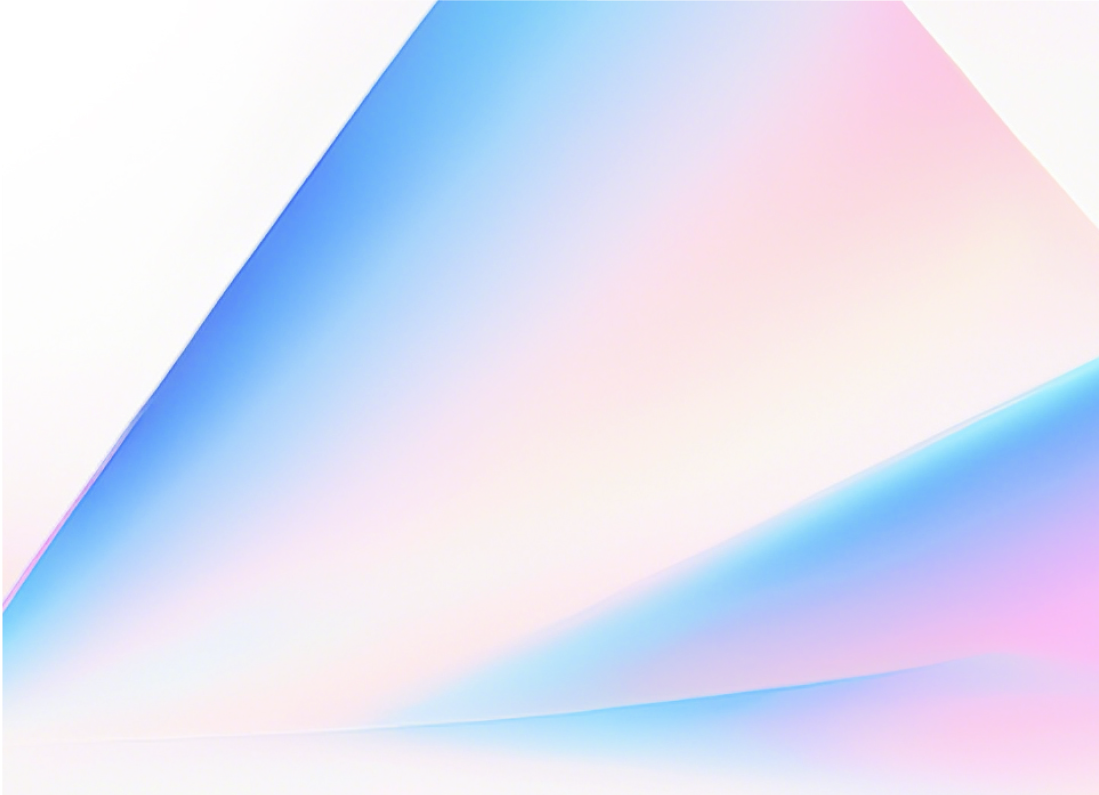
Apr 15, 2025
Seedream 3.0 Technical Report
We present Seedream 3.0, a high-performance Chinese-English bilingual image generation foundation model. We develop several technical improvements to address existing challenges in Seedream 2.0, including alignment with complicated prompts, fine-grained typography generation, suboptimal visual aesthetics and fidelity, and limited image resolutions. Specifically, the advancements of Seedream 3.0 stem from improvements across the entire pipeline, from data construction to model deployment. At the data stratum, we double the dataset using a defect-aware training paradigm and a dual-axis collaborative data-sampling framework. Furthermore, we adopt several effective techniques such as mixed-resolution training, cross-modality RoPE, representation alignment loss, and resolution-aware timestep sampling in the pre-training phase. During the post-training stage, we utilize diversified aesthetic captions in SFT, and a VLM-based reward model with scaling, thereby achieving outputs that well align with human preferences. Furthermore, Seedream 3.0 pioneers a novel acceleration paradigm. By employing consistent noise expectation and importance-aware timestep sampling, we achieve a 4 to 8 times speedup while maintaining image quality. Seedream 3.0 demonstrates significant improvements over Seedream 2.0: it enhances overall capabilities, in particular for text-rendering in complicated Chinese characters which is important to professional typography generation. In addition, it provides native high-resolution output (up to 2K), allowing it to generate images with high visual quality.
Seed Vision Team
Vision
Computer Vision
2025.04.15
Seedream 3.0 Technical Report
Seed Vision Team
Vision
Computer Vision
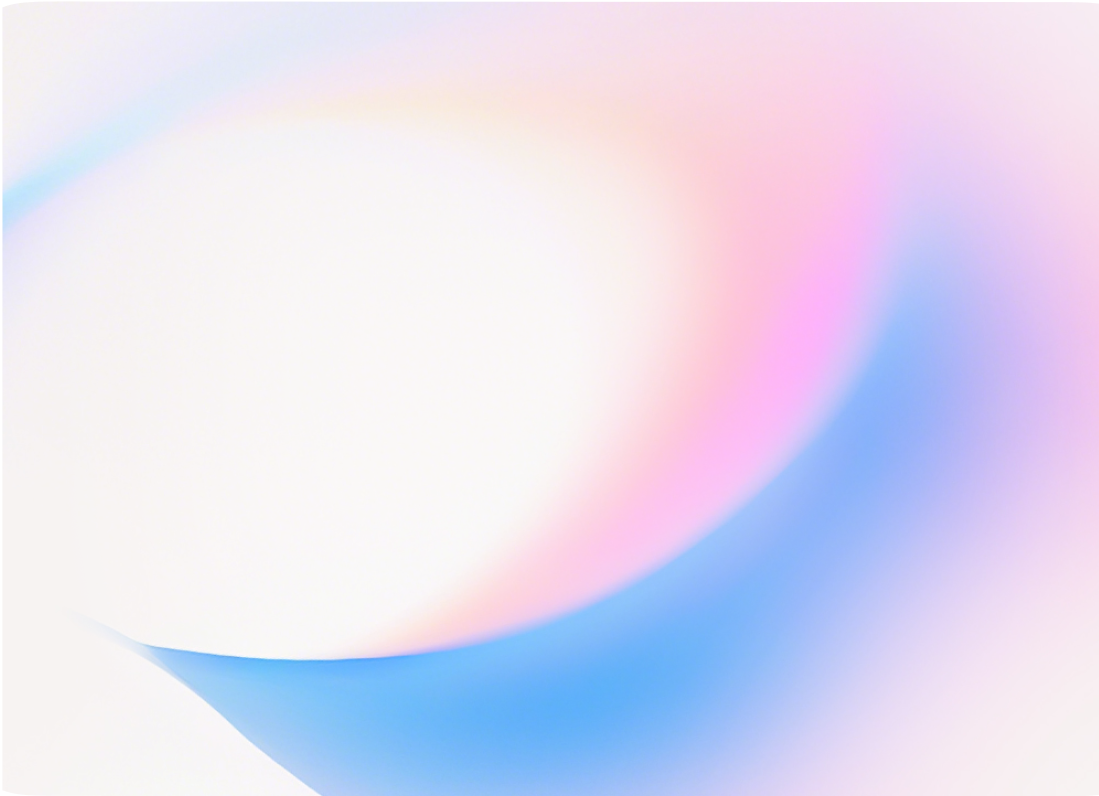
Mar 20, 2025
Multi-Reward as Condition for Instruction-based Image Editing
High-quality training triplets (instruction, original image, edited image) are essential for instruction-based image editing. Predominant training datasets (e.g., InsPix2Pix) are created using text-to-image generative models (e.g., Stable Diffusion, DALL-E) which are not trained for image editing. Accordingly, these datasets suffer from inaccurate instruction following, poor detail preserving, and generation artifacts. In this paper, we propose to address the training data quality issue with multi-perspective reward data instead of refining the ground-truth image quality. 1) we first design a quantitative metric system based on best-in-class LVLM (Large Vision Language Model), i.e., GPT-4o in our case, to evaluate the generation quality from 3 perspectives, namely, instruction following, detail preserving, and generation quality. For each perspective, we collected quantitative score in 0∼5 and text descriptive feedback on the specific failure points in ground-truth edited images, resulting in a high-quality editing reward dataset, i.e., RewardEdit20K. 2) We further proposed a novel training framework to seamlessly integrate the metric output, regarded as multi-reward, into editing models to learn from the imperfect training triplets. During training, the reward scores and text descriptions are encoded as embeddings and fed into both the latent space and the U-Net of the editing models as auxiliary conditions. 3) We also build a challenging evaluation benchmark with real-world images/photos and diverse editing instructions, named Real-Edit. Experiments indicate that our multi-reward conditioned model outperforms its no-reward counterpart on two popular editing pipelines, i.e., InsPix2Pix and SmartEdit. Code is released at this https URL[https://github.com/bytedance/Multi-Reward-Editing].
Xin Gu, Ming Li, Libo Zhang, Fan Chen, Longyin Wen, Tiejian Luo, Sijie Zhu
Vision
Computer Vision
2025.03.20
Multi-Reward as Condition for Instruction-based Image Editing
Xin Gu, Ming Li, Libo Zhang, Fan Chen, Longyin Wen, Tiejian Luo, Sijie Zhu
Vision
Computer Vision
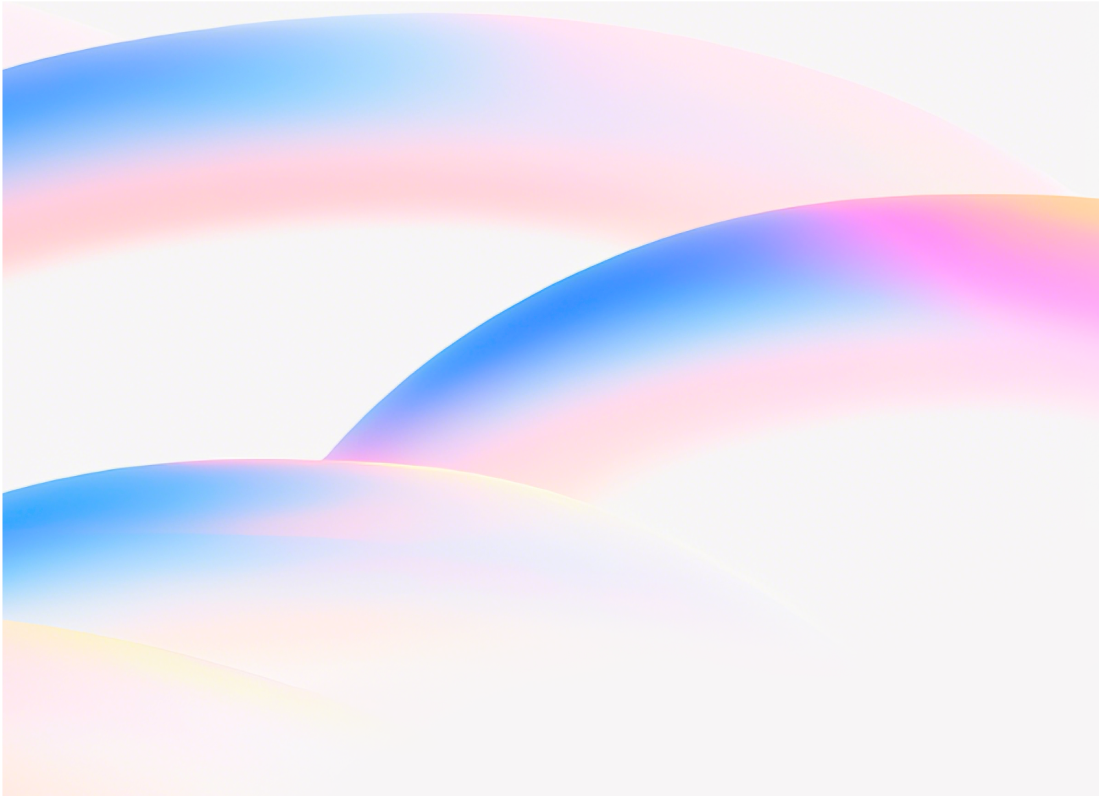
Mar 20, 2025
Polynomial Composition Activations: Unleashing the Dynamics of Large Language Models
Transformers have found extensive applications across various domains due to the powerful fitting capabilities. This success can be partially attributed to their inherent nonlinearity. Thus, in addition to the ReLU function employed in the original transformer architecture, researchers have explored alternative modules such as GeLU and SwishGLU to enhance nonlinearity and thereby augment representational capacity. In this paper, we propose a novel category of polynomial composition activations (PolyCom), designed to optimize the dynamics of transformers. Theoretically, we provide a comprehensive mathematical analysis of PolyCom, highlighting its enhanced expressivity and efficacy relative to other activation functions. Notably, we demonstrate that networks incorporating PolyCom achieve the optimal approximation rate.
Zhijian Zhuo, Ya Wang, Yutao Zeng, Xiaoqing Li, Xun Zhou, Jinwen Ma
Infrastructures
LLM
2025.03.20
Polynomial Composition Activations: Unleashing the Dynamics of Large Language Models
Zhijian Zhuo, Ya Wang, Yutao Zeng, Xiaoqing Li, Xun Zhou, Jinwen Ma
Infrastructures
LLM
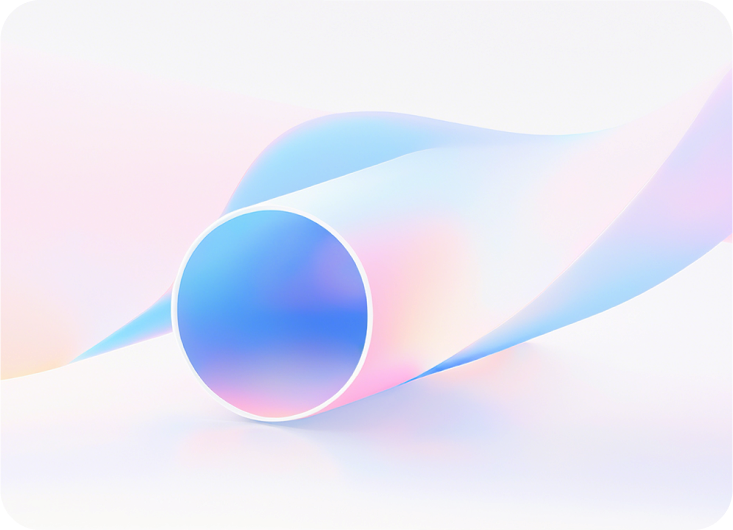
Mar 18, 2025
DAPO: An Open-Source LLM Reinforcement Learning System at Scale
Inference scaling empowers LLMs with unprecedented reasoning ability, with reinforcement learning as the core technique to elicit complex reasoning. However, key technical details of state-of-the-art reasoning LLMs are concealed (such as in OpenAI o1 blog and DeepSeek R1 technical report), thus the community still struggles to reproduce their RL training results.
Qiying Yu, Zheng Zhang, Ruofei Zhu, Yufeng Yuan, Xiaochen Zuo, Yu Yue, Tiantian Fan, Gaohong Liu, Lingjun Liu, Xin Liu, Haibin Lin, Zhiqi Lin, Bole Ma, Guangming Sheng, Yuxuan Tong, Chi Zhang, Mofan Zhang, Wang Zhang, Hang Zhu, Jinhua Zhu, Jiaze Chen, Jiangjie Chen, Chengyi Wang, Hongli Yu, Weinan Dai, Yuxuan Song, Xiangpeng Wei, Hao Zhou, Jingjing Liu, Wei-Ying Ma, Ya-Qin Zhang, Lin Yan, Mu Qiao, Yonghui Wu, Mingxuan Wang
LLM
Reinforcement Learning
2025.03.18
DAPO: An Open-Source LLM Reinforcement Learning System at Scale
Qiying Yu, Zheng Zhang, Ruofei Zhu, Yufeng Yuan, Xiaochen Zuo, Yu Yue, Tiantian Fan, Gaohong Liu, Lingjun Liu, Xin Liu, Haibin Lin, Zhiqi Lin, Bole Ma, Guangming Sheng, Yuxuan Tong, Chi Zhang, Mofan Zhang, Wang Zhang, Hang Zhu, Jinhua Zhu, Jiaze Chen, Jiangjie Chen, Chengyi Wang, Hongli Yu, Weinan Dai, Yuxuan Song, Xiangpeng Wei, Hao Zhou, Jingjing Liu, Wei-Ying Ma, Ya-Qin Zhang, Lin Yan, Mu Qiao, Yonghui Wu, Mingxuan Wang
LLM
Reinforcement Learning
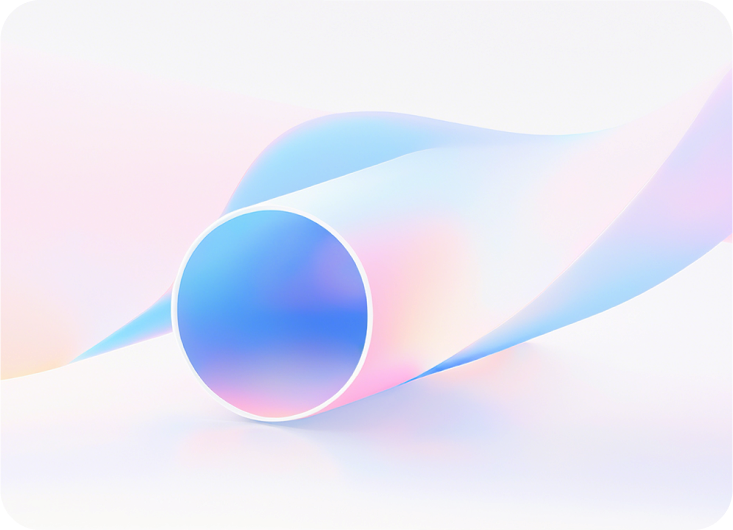
Mar 18, 2025
Hyper-Connections
We present hyper-connections, a simple yet effective method that can serve as an alternative to residual connections. This approach specifically addresses common drawbacks observed in residual connection variants, such as the seesaw effect between gradient vanishing and representation collapse. Theoretically, hyper-connections allow the network to adjust the strength of connections between features at different depths and dynamically rearrange layers. We conduct experiments focusing on the pre-training of large language models, including dense and sparse models, where hyper-connections show significant performance improvements over residual connections. Additional experiments conducted on vision tasks also demonstrate similar improvements. We anticipate that this method will be broadly applicable and beneficial across a wide range of AI problems.
Defa Zhu, Hongzhi Huang, Zihao Huang, Yutao Zeng, Yunyao Mao, Banggu Wu, Qiyang Min, Xun Zhou
Infrastructures
LLM
2025.03.18
Hyper-Connections
Defa Zhu, Hongzhi Huang, Zihao Huang, Yutao Zeng, Yunyao Mao, Banggu Wu, Qiyang Min, Xun Zhou
Infrastructures
LLM
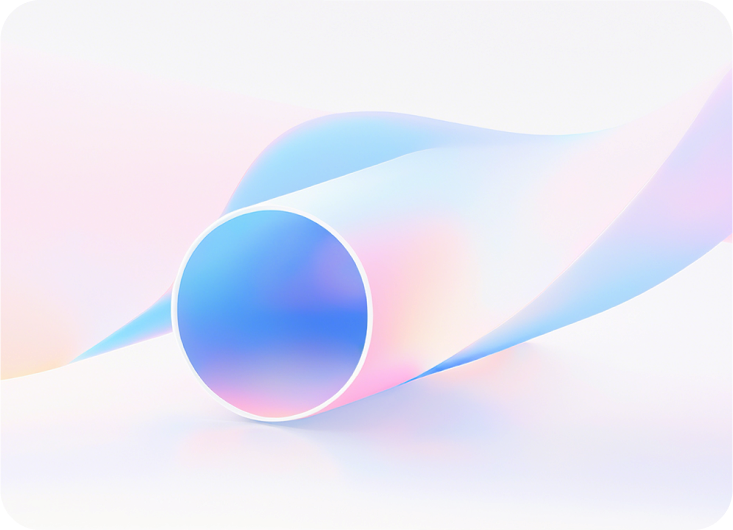
Mar 17, 2025
FlexWorld: Progressively Expanding 3D Scenes for Flexiable-View Synthesis
Generating flexible-view 3D scenes, including 360° rotation and zooming, from single images is challenging due to a lack of 3D data. To this end, we introduce FlexWorld, a novel framework consisting of two key components: (1) a strong video-to-video (V2V) diffusion model to generate high-quality novel view images from incomplete input rendered from a coarse scene, and (2) a progressive expansion process to construct a complete 3D scene. In particular, leveraging an advanced pre-trained video model and accurate depth-estimated training pairs, our V2V model can generate novel views under large camera pose variations. Building upon it, FlexWorld progressively generates new 3D content and integrates it into the global scene through geometry-aware scene fusion. Extensive experiments demonstrate the effectiveness of FlexWorld in generating high-quality novel view videos and flexible-view 3D scenes from single images, achieving superior visual quality under multiple popular metrics and datasets compared to existing state-of-the-art methods. Qualitatively, we highlight that FlexWorld can generate high-fidelity scenes with flexible views like 360° rotations and zooming.
Luxi Chen, Zihan Zhou, Min Zhao, Yikai Wang, Ge Zhang, Wenhao Huang, Hao Sun, Ji-Rong Wen, Chongxuan Li
Vision
Computer Vision
2025.03.17
FlexWorld: Progressively Expanding 3D Scenes for Flexiable-View Synthesis
Luxi Chen, Zihan Zhou, Min Zhao, Yikai Wang, Ge Zhang, Wenhao Huang, Hao Sun, Ji-Rong Wen, Chongxuan Li
Vision
Computer Vision
View more