Speech
Seed-语音团队的使命是利用多模态语音技术丰富交互和创作方式。团队专注于语音和音频、音乐、自然语言理解和多模态深度学习等领域的前沿研究和产品创新
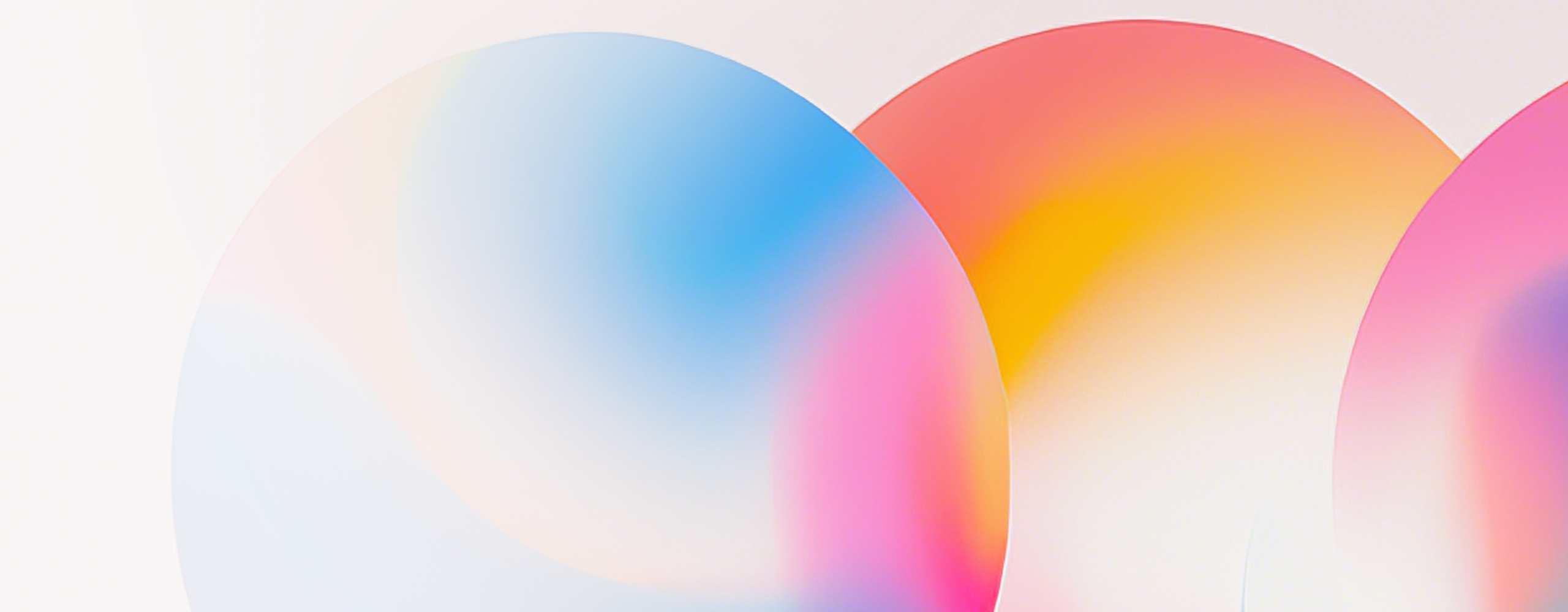
课题方向
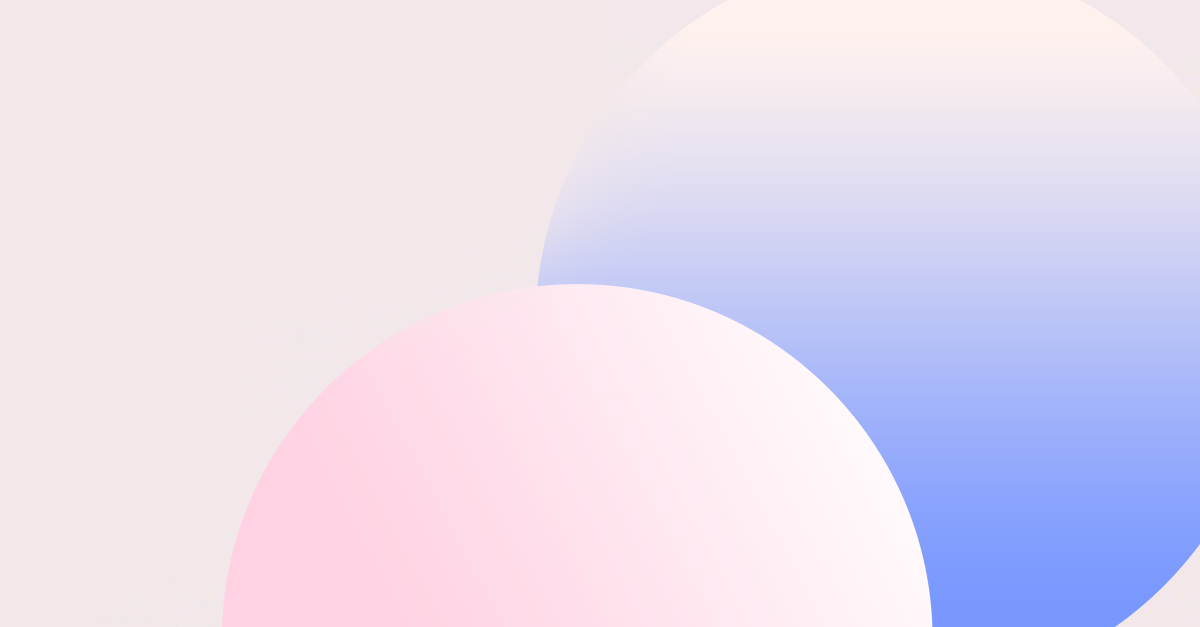
音频及音乐理解生成基座大模型
音频理解和生成基座大模型,探索语音识别、合成、转换、音乐生成、音效生成的统一建模方式
AI foundation
Audio
AI foundation
Audio
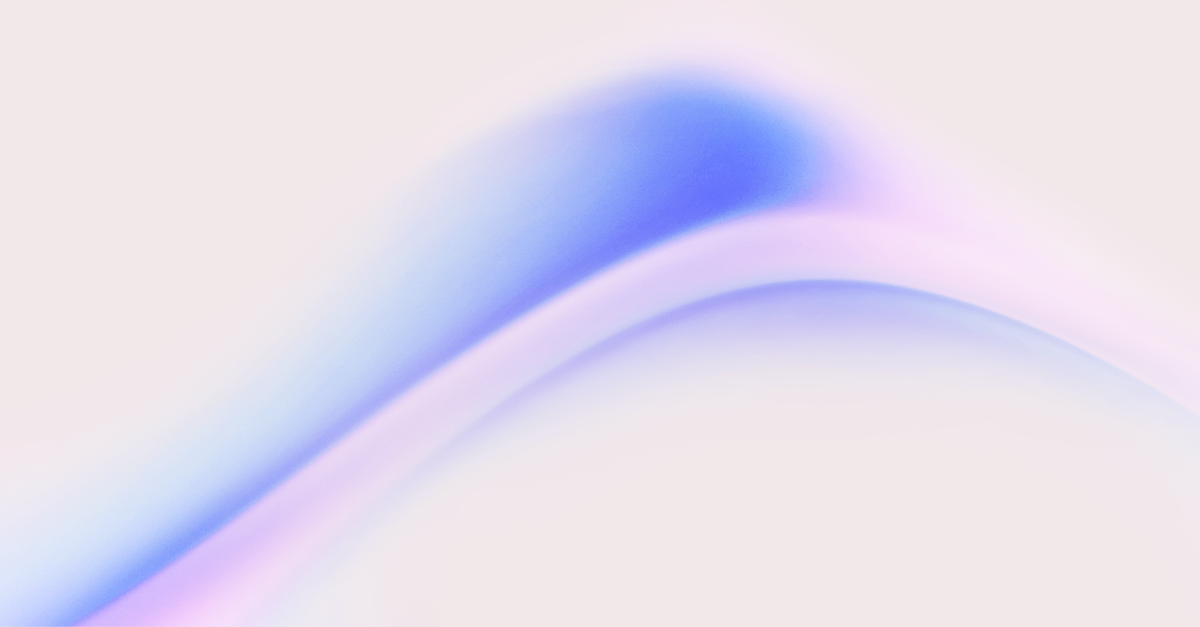
多模态模型设计和优化
多模态模型网络结构设计和优化、扩散模型的设计和优化
Multimodal
Optimization
Multimodal
Optimization
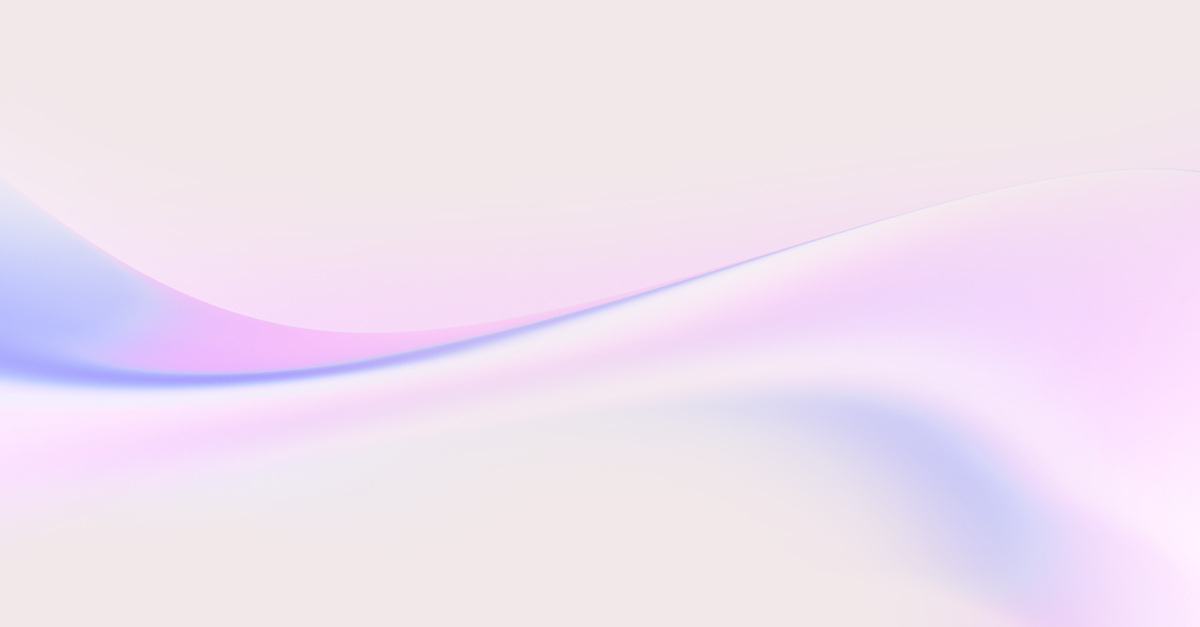
强化学习在音频场景下的应用
强化学习在语音/音频多模态大模型场景下的应用,以及 RL 系统方案设计和优化
Reinforcement learning
Application
Reinforcement learning
Application
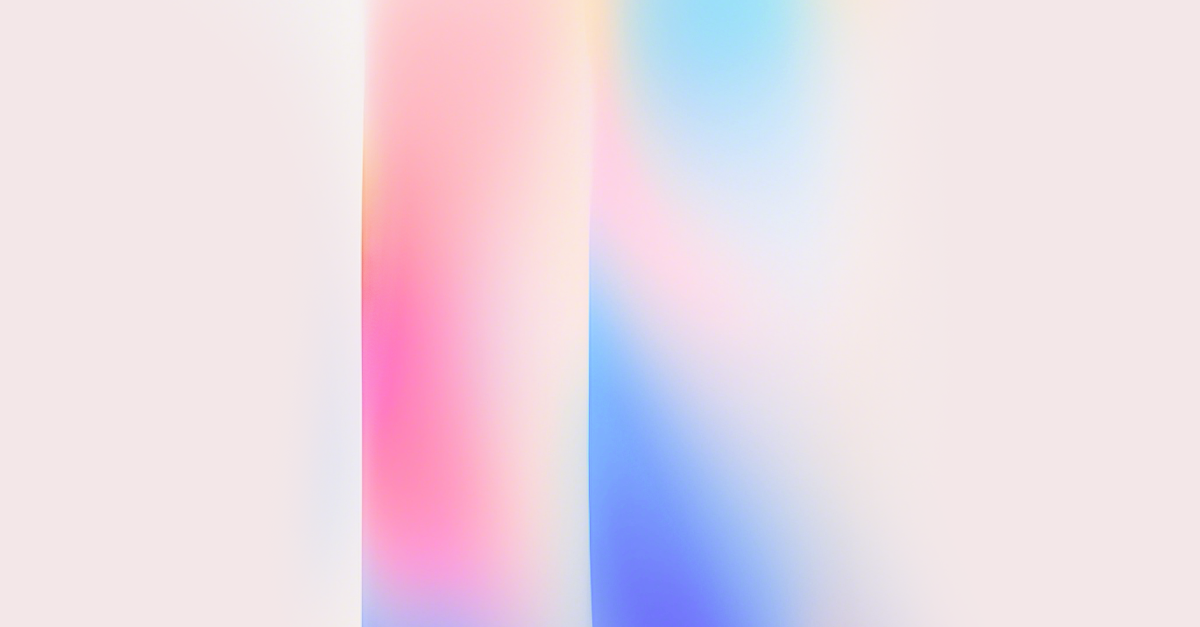
大规模分布式训练推理系统
探索高效的大规模分布式训练和推理系统
Large-scale
System
Large-scale
System
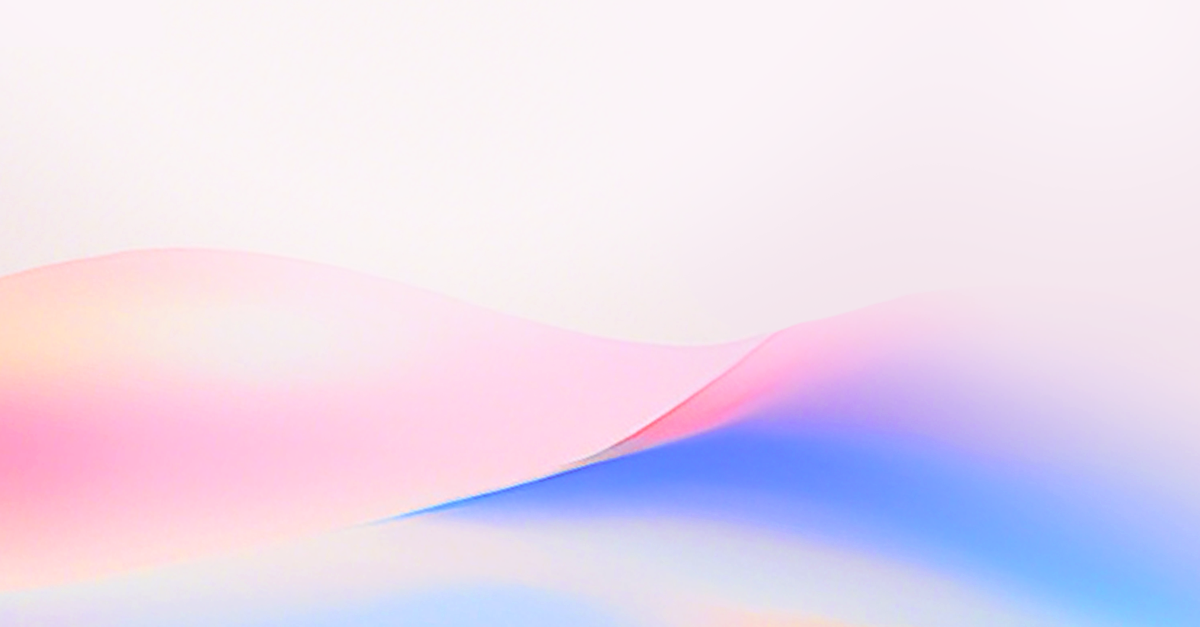
语音场景下的机器学习平台建设
高可用、可扩展、分布式机器学习平台的建设,支撑语音/音频相关算法生产与高效迭代
Machine learning
Audio
Machine learning
Audio
精选论文
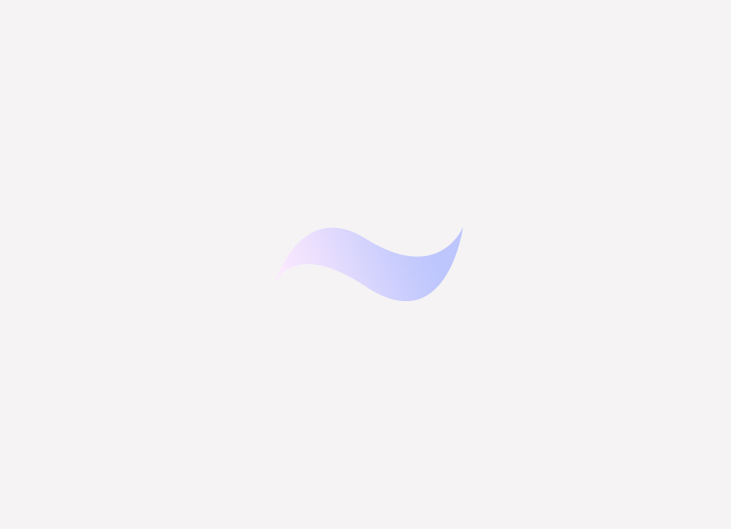
2025.02.25
You Only Sample Once: Taming One-Step Text-to-Image Synthesis by Self-Cooperative Diffusion GANs
Recently, some works have tried to combine diffusion and Generative Adversarial Networks (GANs) to alleviate the computational cost of the iterative denoising inference in Diffusion Models (DMs). However, existing works in this line suffer from either training instability and mode collapse or subpar one-step generation learning efficiency. To address these issues, we introduce YOSO, a novel generative model designed for rapid, scalable, and high-fidelity one-step image synthesis with high training stability and mode coverage. Specifically, we smooth the adversarial divergence by the denoising generator itself, performing self-cooperative learning. We show that our method can serve as a one-step generation model training from scratch with competitive performance. Moreover, we extend our YOSO to one-step text-to-image generation based on pre-trained models by several effective training techniques (i.e., latent perceptual loss and latent discriminator for efficient training along with the latent DMs; the informative prior initialization (IPI), and the quick adaption stage for fixing the flawed noise scheduler). Experimental results show that YOSO achieves the state-of-the-art one-step generation performance even with Low-Rank Adaptation (LoRA) fine-tuning. In particular, we show that the YOSO-PixArt-α can generate images in one step trained on 512 resolution, with the capability of adapting to 1024 resolution without extra explicit training, requiring only ~10 A800 days for fine-tuning. Our code is provided at this https URL[https://github.com/Luo-Yihong/YOSO].
Yihong Luo, Xiaolong Chen, Xinghua Qu, Tianyang Hu, Jing Tang
Computer Vision
2025.02.25
You Only Sample Once: Taming One-Step Text-to-Image Synthesis by Self-Cooperative Diffusion GANs
Yihong Luo, Xiaolong Chen, Xinghua Qu, Tianyang Hu, Jing Tang
Computer Vision
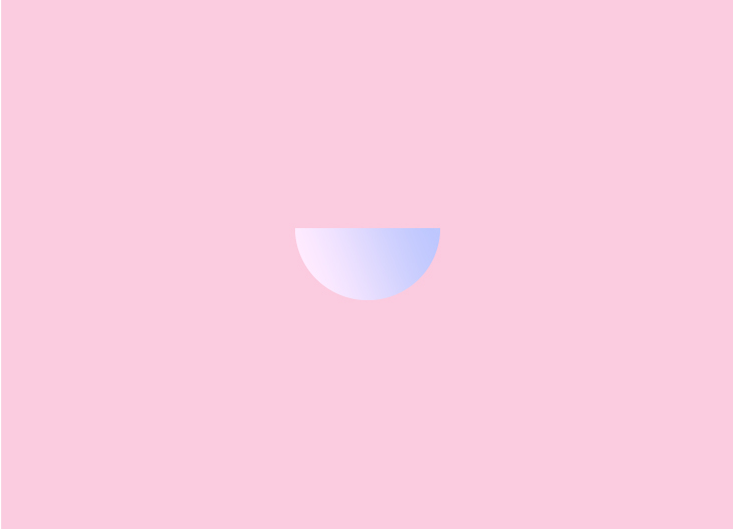
2024.09.13
Seed-Music: A Unified Framework for High Quality and Controlled Music Generation
We introduce Seed-Music, a suite of music generation systems capable of producing high-quality music with fine-grained style control. Our unified framework leverages both auto-regressive language modeling and diffusion approaches to support two key music creation workflows: controlled music generation and postproduction editing. For controlled music generation, our system enables vocal music generation with performance controls from multi-modal inputs, including style descriptions, audio references, musical scores, and voice prompts. For postproduction editing, it offers interactive tools for editing lyrics and vocal melodies directly in the generated audio.
We encourage readers to listen to demo audio examples at https://team.doubao.com/seed-music.
Ye Bai, Haonan Chen, Jitong Chen, Zhuo Chen, Yi Deng, Xiaohong Dong, Lamtharn Hantrakul, Weituo Hao, Qingqing Huang, Zhongyi Huang, Dongya Jia, Feihu La, Duc Le, Bochen Li, Chumin Li, Hui Li, Xingxing Li, Shouda Liu, Wei-Tsung Lu, Yiqing Lu, Andrew Shaw, Janne Spijkervet, Yakun Sun, Bo Wang, Ju-Chiang Wang, Yuping Wang, Yuxuan Wang, Ling Xu, Yifeng Yang, Chao Yao, Shuo Zhang, Yang Zhang, Yilin Zhang, Hang Zhao, Ziyi Zhao, Dejian Zhong, Shicen Zhou, Pei Zou
Speech&Audio
2024.09.13
Seed-Music: A Unified Framework for High Quality and Controlled Music Generation
Ye Bai, Haonan Chen, Jitong Chen, Zhuo Chen, Yi Deng, Xiaohong Dong, Lamtharn Hantrakul, Weituo Hao, Qingqing Huang, Zhongyi Huang, Dongya Jia, Feihu La, Duc Le, Bochen Li, Chumin Li, Hui Li, Xingxing Li, Shouda Liu, Wei-Tsung Lu, Yiqing Lu, Andrew Shaw, Janne Spijkervet, Yakun Sun, Bo Wang, Ju-Chiang Wang, Yuping Wang, Yuxuan Wang, Ling Xu, Yifeng Yang, Chao Yao, Shuo Zhang, Yang Zhang, Yilin Zhang, Hang Zhao, Ziyi Zhao, Dejian Zhong, Shicen Zhou, Pei Zou
Speech&Audio
查看更多论文
技术能力展示
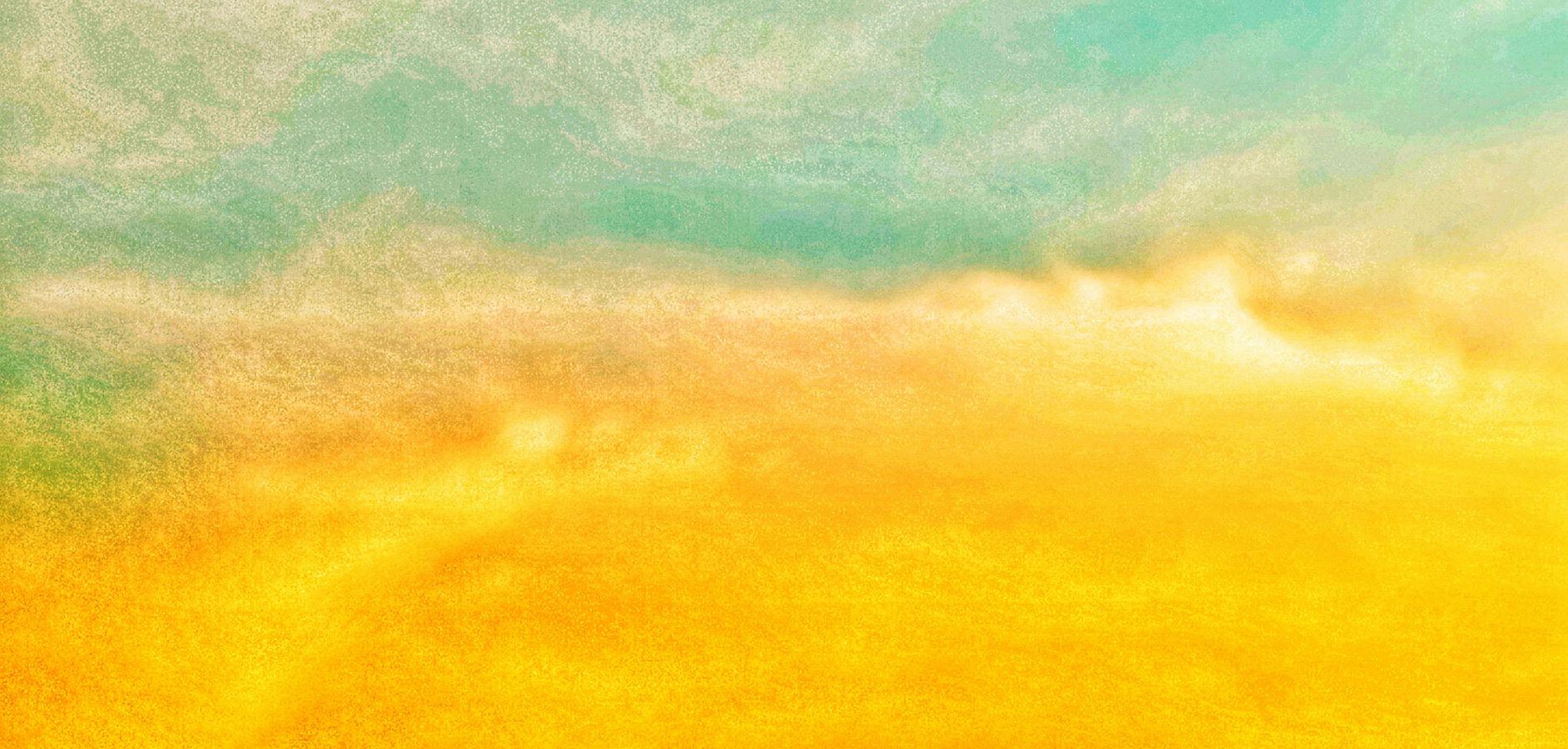
Seed Realtime Voice Model
Seed Realtime Voice Model 实时语音大模型,可实现真人级别的端到端语音对话交互。相比传统级联模式,在语音表现力、控制力、情绪承接方面表现惊艳,并具备低时延、对话中可随时打断等特性。
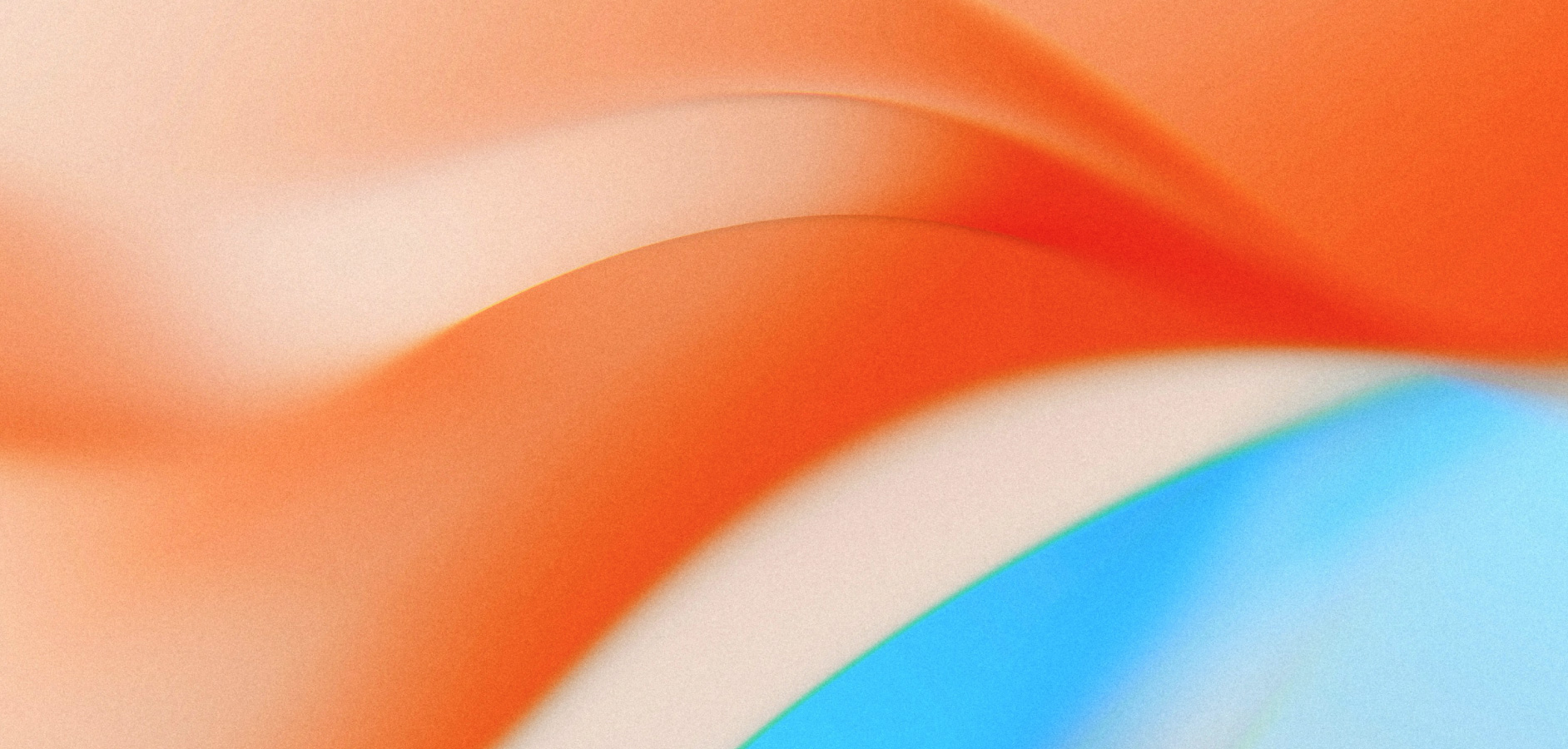
Seed-Music
Seed-Music 是一个具有灵活控制能力的音乐生成模型家族,提供了可控音乐生成、谱转曲、词曲编辑、零样本人声克隆四大核心功能,融合了语言模型和扩散模型优势,融入作曲工作流。
热招岗位
语音机器学习平台开发工程师-Seed
大模型数据工程师-Seed
高性能计算研发工程师-Seed
音视频多模态算法工程师-Seed
音频多模态算法研究实习生-Top Seed Intern