Vision
Seed-视觉团队致力于视觉生成的基础模型、多模态生成模型、以及基于生成式 AI 视觉基础问题的前沿科研和应用研发
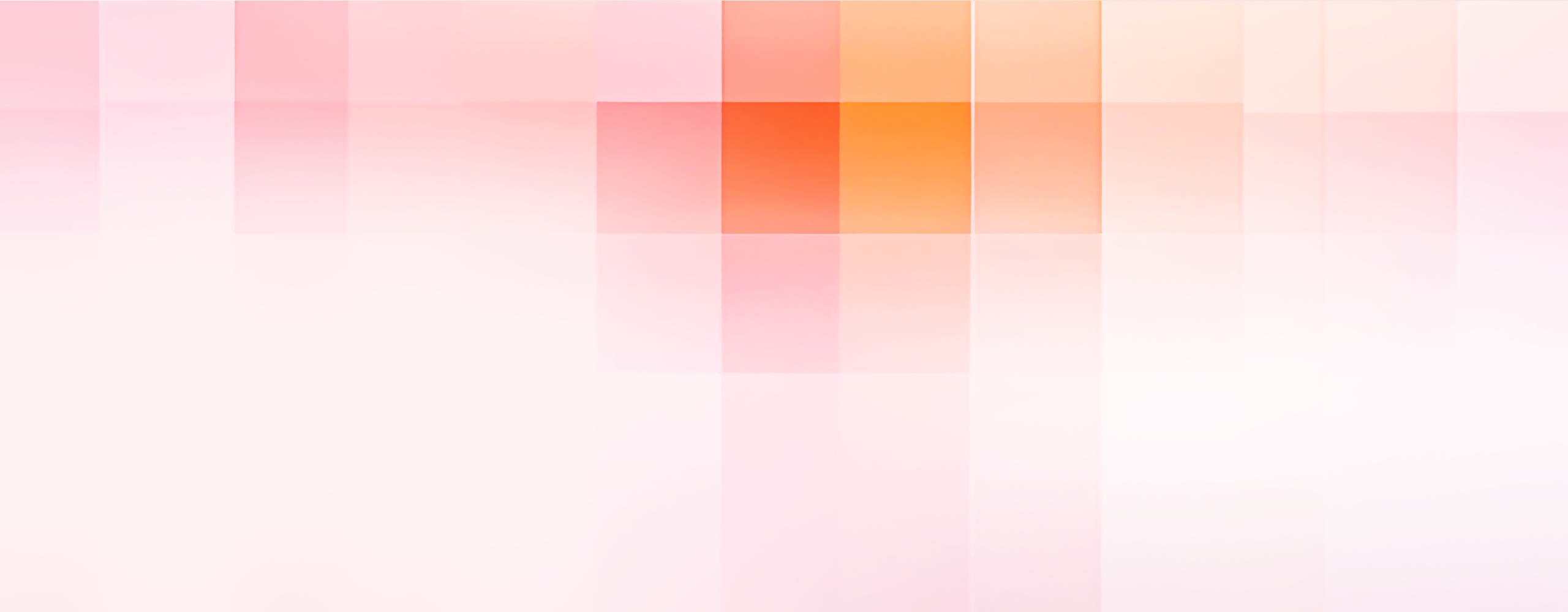
研究领域
研究方向
团队专注于视觉生成模型,多模态架构,以及人工智能视觉相关领域的技术研究
探索课题
包括 AIGC、扩散模型、自回归模型、多模态模型、3D/4D 生成、视觉自监督学习、模型优化加速等
课题方向
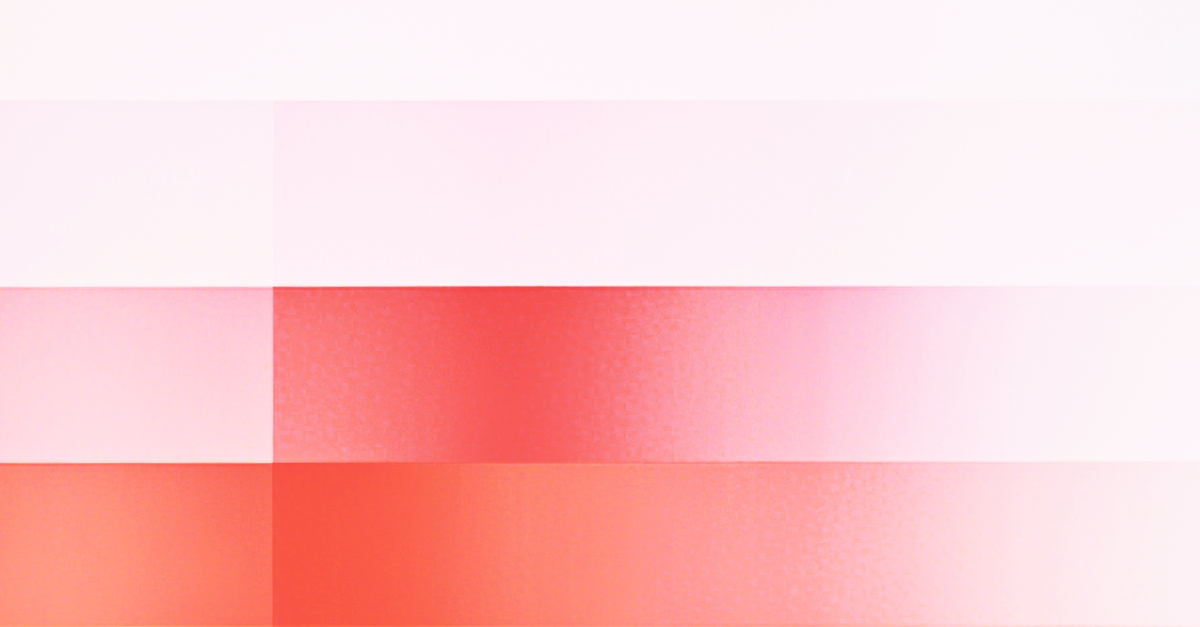
视觉生成基础模型
研发视觉生成(图像和视频)的基座模型,提供视觉生成高交互性和高可控性,理解视频中的视觉规律,探索基于生成基座模型的各种视觉任务
Multimodal
Diffusion Model
Auto Regression Model
Foundation
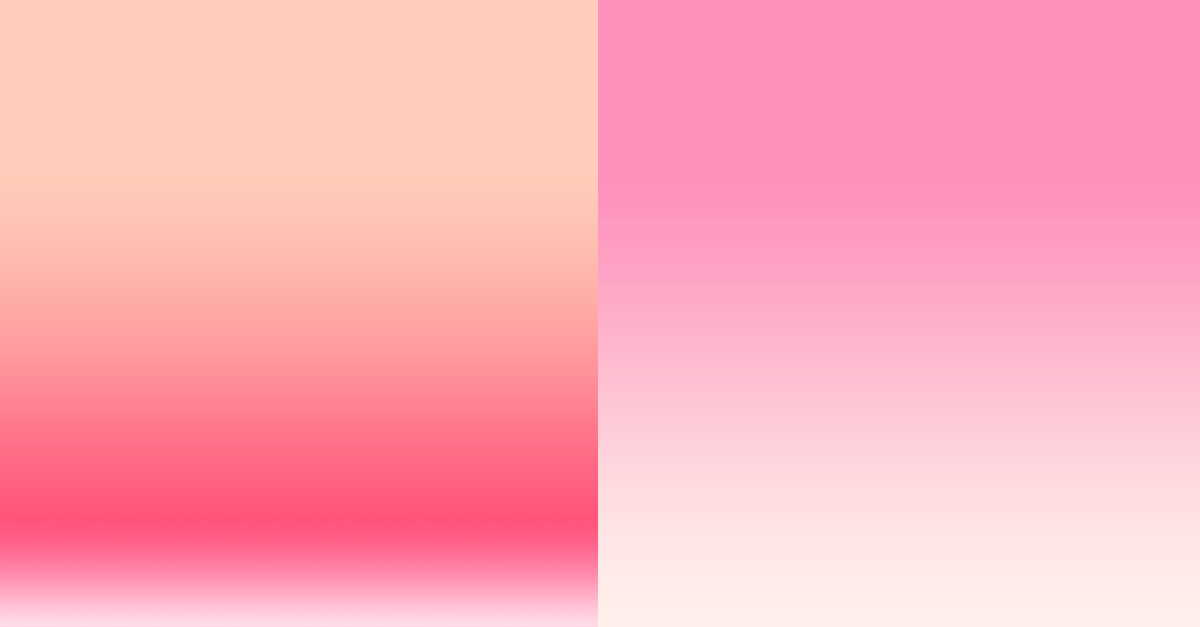
多模态生成模型
融合多种模态的统一生成模型,生成和理解联合建模,支持多模态的交织生成和同时生成(E.g. 数字人),提升生成模型上下文能力和一致性
Multimodel
Diffusion Model
Auto Regression Model
Foundation
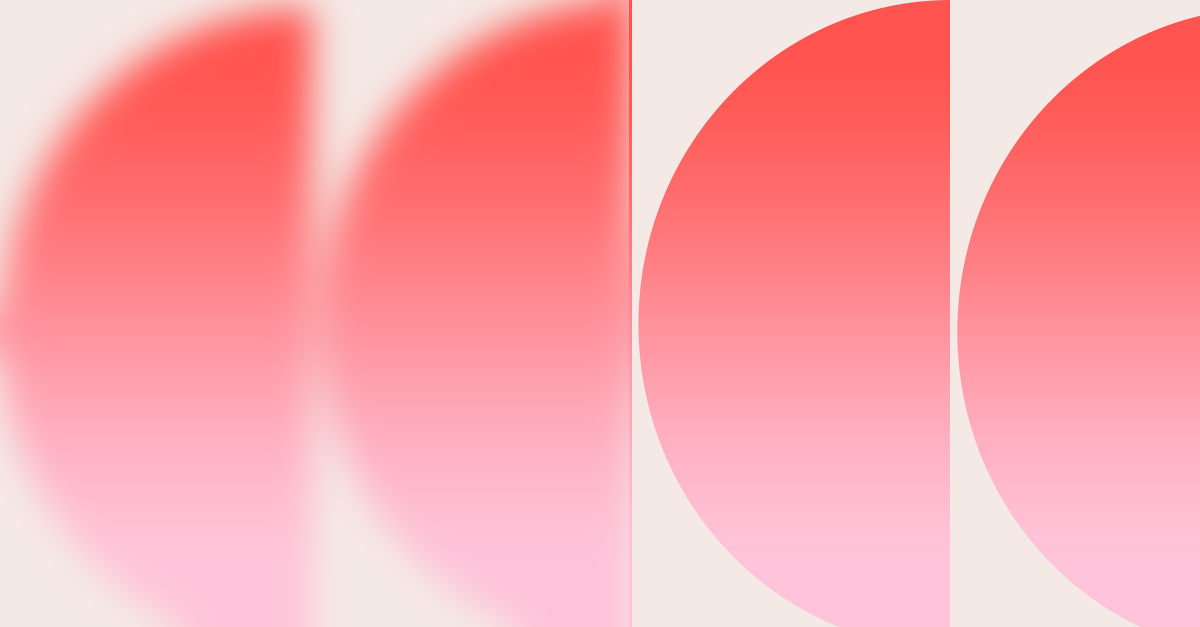
3D/4D 生成模型
3D/4D 生成基础模型,从视频数据和 3D 数据学习视觉世界知识,理解物理世界 3D 空间和物理规律,构建视觉的空间智能和世界模型,探索基于生成模型的物理和渲染引擎
3D
4D
World Model
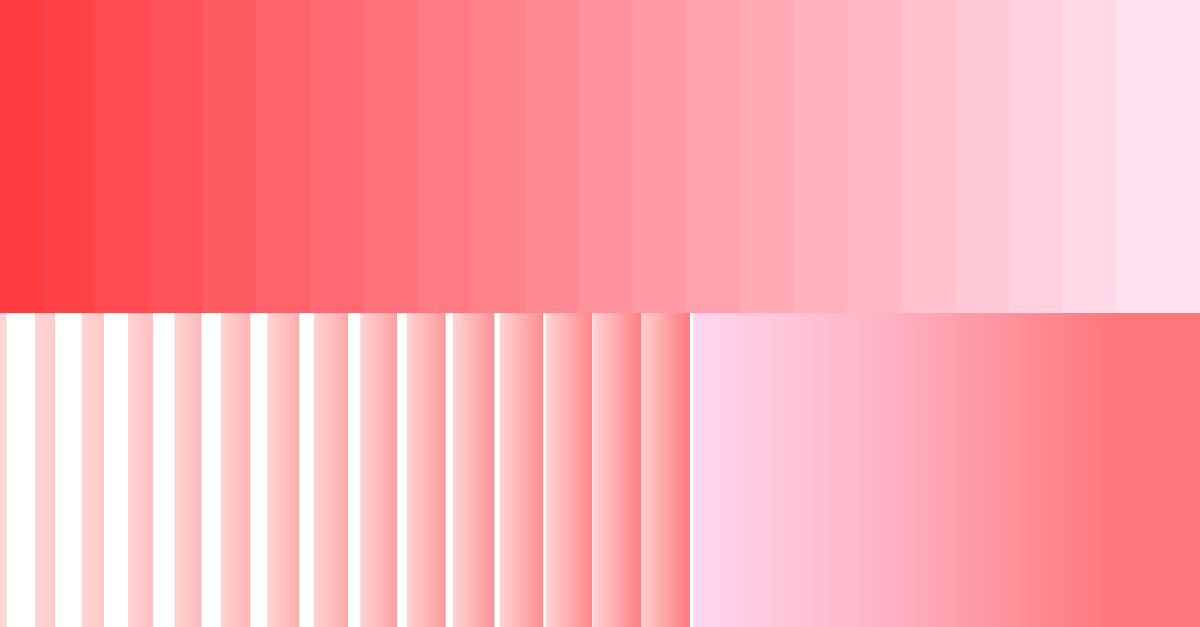
多模态模型设计和优化
多模态模型网络架构设计和优化、扩散模型的优化、高效的大规模分布式训练和推理、模型加速和优化
Multimodal
Optimization
Distillation
Quantization
精选论文
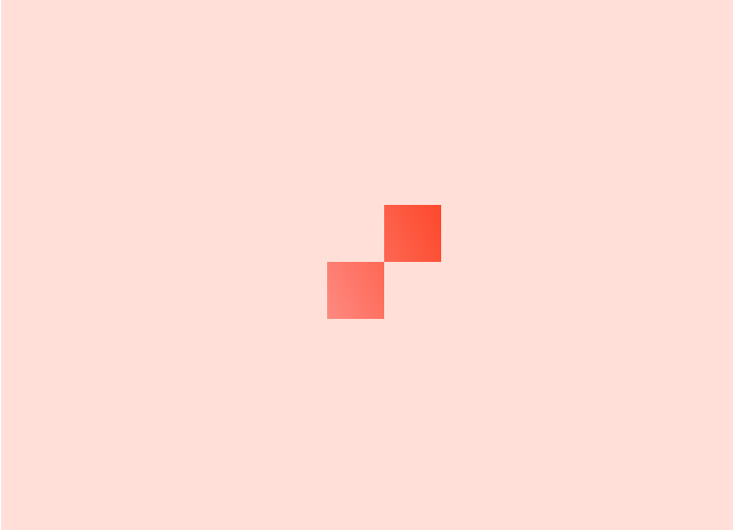
2025.04.15
Seedream 3.0 Technical Report
We present Seedream 3.0, a high-performance Chinese-English bilingual image generation foundation model. We develop several technical improvements to address existing challenges in Seedream 2.0, including alignment with complicated prompts, fine-grained typography generation, suboptimal visual aesthetics and fidelity, and limited image resolutions. Specifically, the advancements of Seedream 3.0 stem from improvements across the entire pipeline, from data construction to model deployment. At the data stratum, we double the dataset using a defect-aware training paradigm and a dual-axis collaborative data-sampling framework. Furthermore, we adopt several effective techniques such as mixed-resolution training, cross-modality RoPE, representation alignment loss, and resolution-aware timestep sampling in the pre-training phase. During the post-training stage, we utilize diversified aesthetic captions in SFT, and a VLM-based reward model with scaling, thereby achieving outputs that well align with human preferences. Furthermore, Seedream 3.0 pioneers a novel acceleration paradigm. By employing consistent noise expectation and importance-aware timestep sampling, we achieve a 4 to 8 times speedup while maintaining image quality. Seedream 3.0 demonstrates significant improvements over Seedream 2.0: it enhances overall capabilities, in particular for text-rendering in complicated Chinese characters which is important to professional typography generation. In addition, it provides native high-resolution output (up to 2K), allowing it to generate images with high visual quality.
Seed Vision Team
Computer Vision
2025.04.15
Seedream 3.0 Technical Report
Seed Vision Team
Vision
Computer Vision
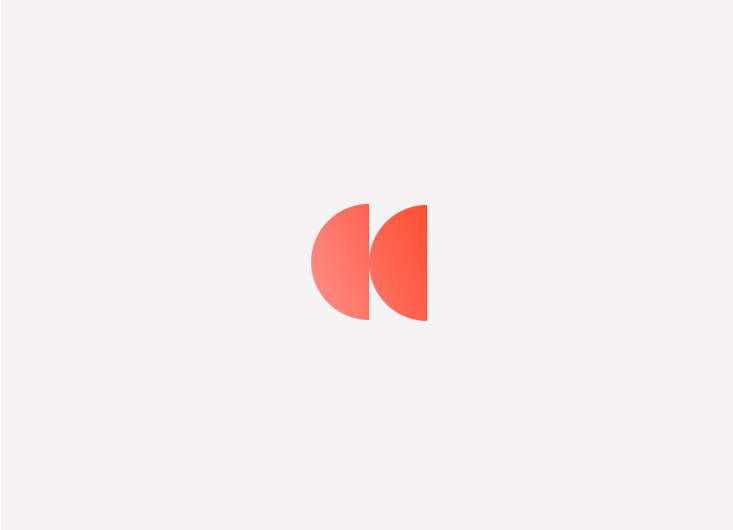
2025.03.20
Multi-Reward as Condition for Instruction-based Image Editing
High-quality training triplets (instruction, original image, edited image) are essential for instruction-based image editing. Predominant training datasets (e.g., InsPix2Pix) are created using text-to-image generative models (e.g., Stable Diffusion, DALL-E) which are not trained for image editing. Accordingly, these datasets suffer from inaccurate instruction following, poor detail preserving, and generation artifacts. In this paper, we propose to address the training data quality issue with multi-perspective reward data instead of refining the ground-truth image quality. 1) we first design a quantitative metric system based on best-in-class LVLM (Large Vision Language Model), i.e., GPT-4o in our case, to evaluate the generation quality from 3 perspectives, namely, instruction following, detail preserving, and generation quality. For each perspective, we collected quantitative score in 0∼5 and text descriptive feedback on the specific failure points in ground-truth edited images, resulting in a high-quality editing reward dataset, i.e., RewardEdit20K. 2) We further proposed a novel training framework to seamlessly integrate the metric output, regarded as multi-reward, into editing models to learn from the imperfect training triplets. During training, the reward scores and text descriptions are encoded as embeddings and fed into both the latent space and the U-Net of the editing models as auxiliary conditions. 3) We also build a challenging evaluation benchmark with real-world images/photos and diverse editing instructions, named Real-Edit. Experiments indicate that our multi-reward conditioned model outperforms its no-reward counterpart on two popular editing pipelines, i.e., InsPix2Pix and SmartEdit. Code is released at this https URL[https://github.com/bytedance/Multi-Reward-Editing].
Xin Gu, Ming Li, Libo Zhang, Fan Chen, Longyin Wen, Tiejian Luo, Sijie Zhu
Computer Vision
2025.03.20
Multi-Reward as Condition for Instruction-based Image Editing
Xin Gu, Ming Li, Libo Zhang, Fan Chen, Longyin Wen, Tiejian Luo, Sijie Zhu
Vision
Computer Vision
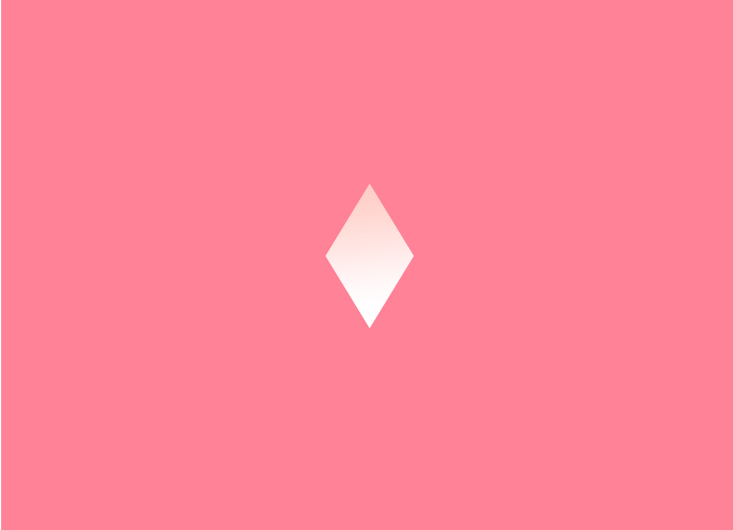
2025.03.17
FlexWorld: Progressively Expanding 3D Scenes for Flexiable-View Synthesis
Generating flexible-view 3D scenes, including 360° rotation and zooming, from single images is challenging due to a lack of 3D data. To this end, we introduce FlexWorld, a novel framework consisting of two key components: (1) a strong video-to-video (V2V) diffusion model to generate high-quality novel view images from incomplete input rendered from a coarse scene, and (2) a progressive expansion process to construct a complete 3D scene. In particular, leveraging an advanced pre-trained video model and accurate depth-estimated training pairs, our V2V model can generate novel views under large camera pose variations. Building upon it, FlexWorld progressively generates new 3D content and integrates it into the global scene through geometry-aware scene fusion. Extensive experiments demonstrate the effectiveness of FlexWorld in generating high-quality novel view videos and flexible-view 3D scenes from single images, achieving superior visual quality under multiple popular metrics and datasets compared to existing state-of-the-art methods. Qualitatively, we highlight that FlexWorld can generate high-fidelity scenes with flexible views like 360° rotations and zooming.
Luxi Chen, Zihan Zhou, Min Zhao, Yikai Wang, Ge Zhang, Wenhao Huang, Hao Sun, Ji-Rong Wen, Chongxuan Li
Computer Vision
2025.03.17
FlexWorld: Progressively Expanding 3D Scenes for Flexiable-View Synthesis
Luxi Chen, Zihan Zhou, Min Zhao, Yikai Wang, Ge Zhang, Wenhao Huang, Hao Sun, Ji-Rong Wen, Chongxuan Li
Vision
Computer Vision
查看更多论文
技术应用
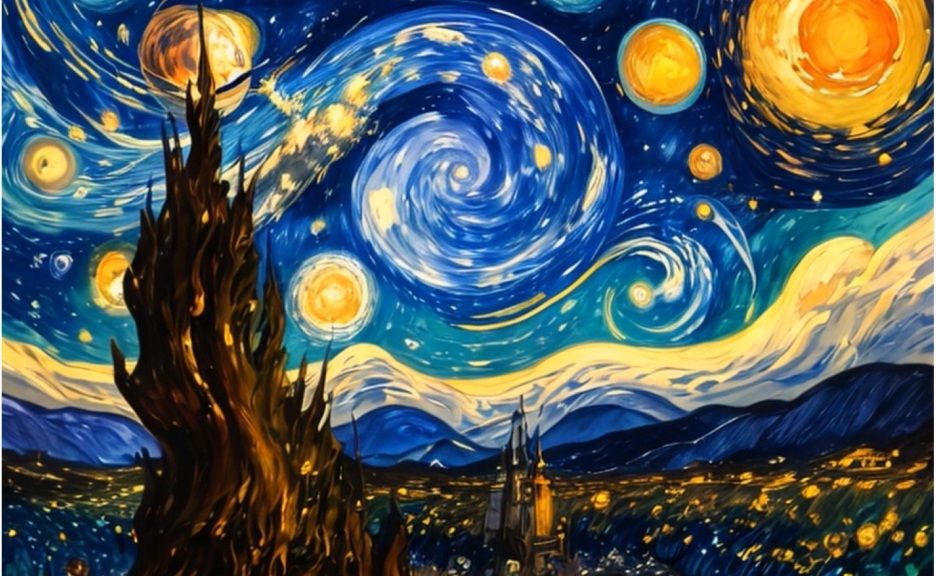
豆包·文生图模型
豆包·文生图模型现已应用于抖音、剪映、豆包、星绘等产品。在豆包 App 中输入提示词,即可生成兼具光影明暗、氛围色彩和人物美感的高质量图像,同时支持中英文双语输入,对复杂 prompt 的理解同样精准。
Text-to-Image
Model
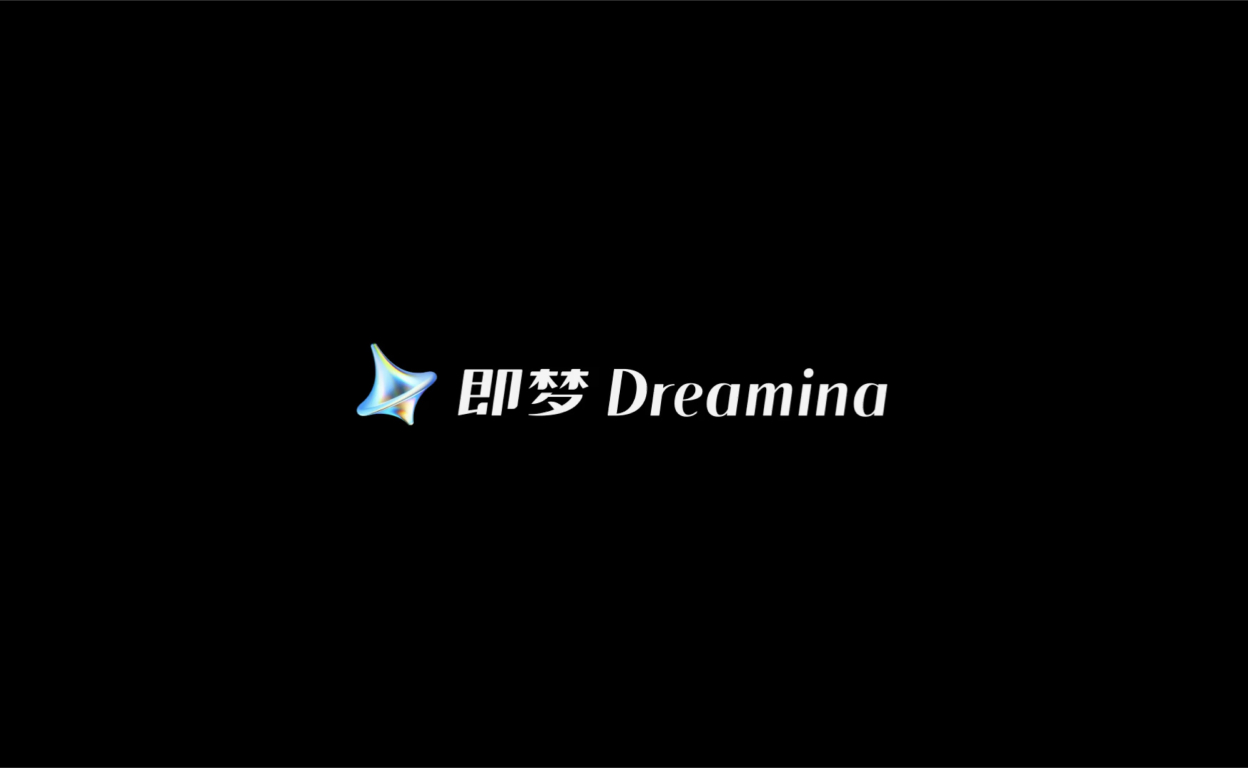
即梦
即梦是一款由字节跳动自主研发的 AI 创作产品,支持通过自然语言及图片输入,生成高质量的图像及视频。平台提供智能画布、故事创作模式及各种 AI 编辑能力,为用户的创作提效。
AI-powered
Creative