Vision
The Seed Vision team focuses on foundational models for visual generation, developing multimodal generative models, and carrying out leading research and application development to solve fundamental computer vision challenges in GenAI.
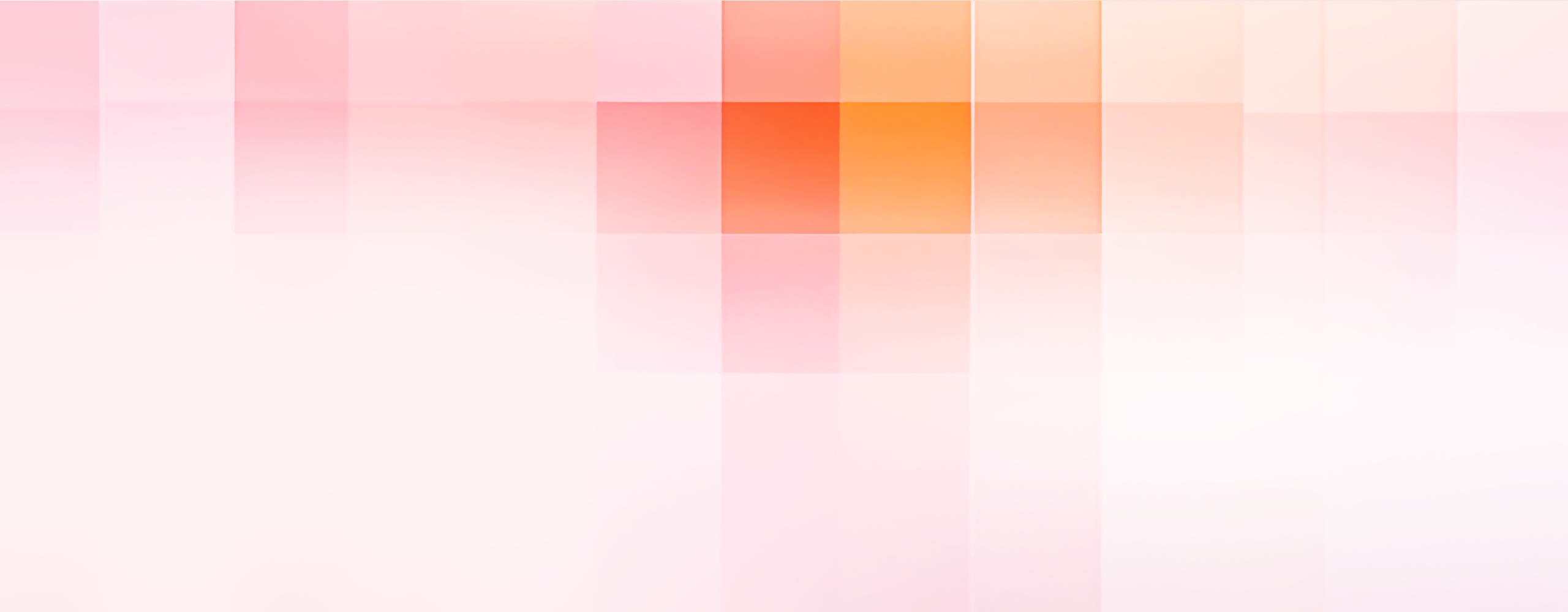
Main areas of focus
Research focus
The team focuses on visual generation models, multimodal architectures, and technology research in AI vision-related areas.
Areas for exploration
This includes AIGC, diffusion models, autoregressive models, multimodal models, 3D/4D generation, visual self-supervised learning, and accelerating model optimization.
Research topics
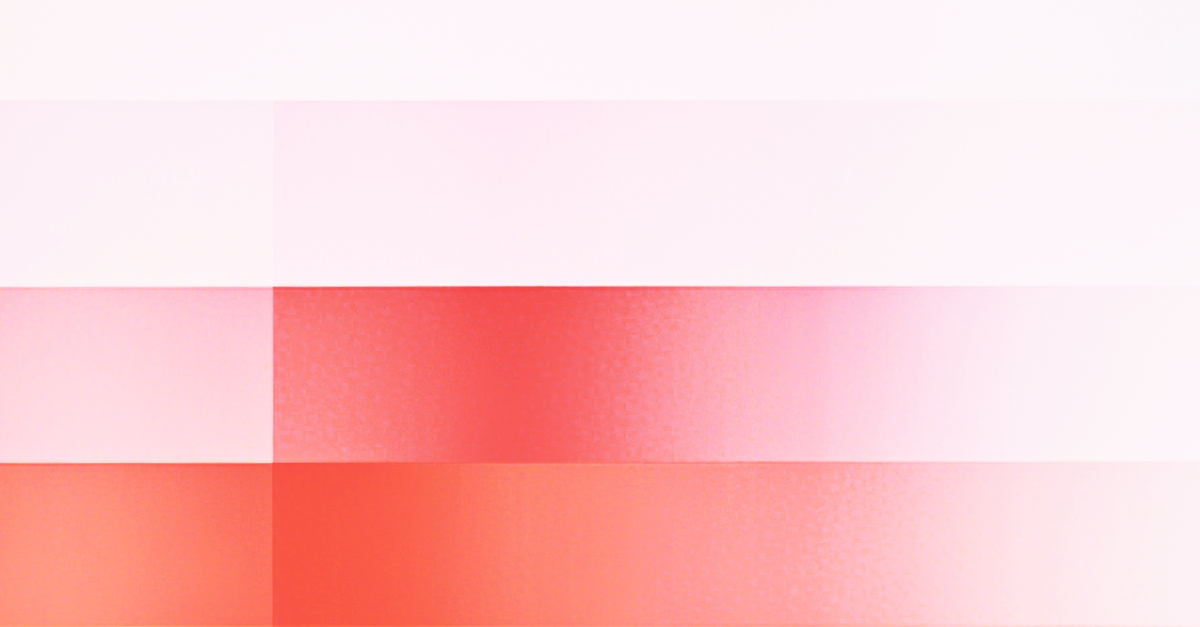
Foundational models for visual generation
Researching and developing foundational models for visual generation (images and videos), ensuring high interactivity and controllability in visual generation, understanding patterns in videos, and exploring various visual-oriented tasks based on generative foundational models.
Multimodal
Diffusion Model
Auto Regression Model
Foundation
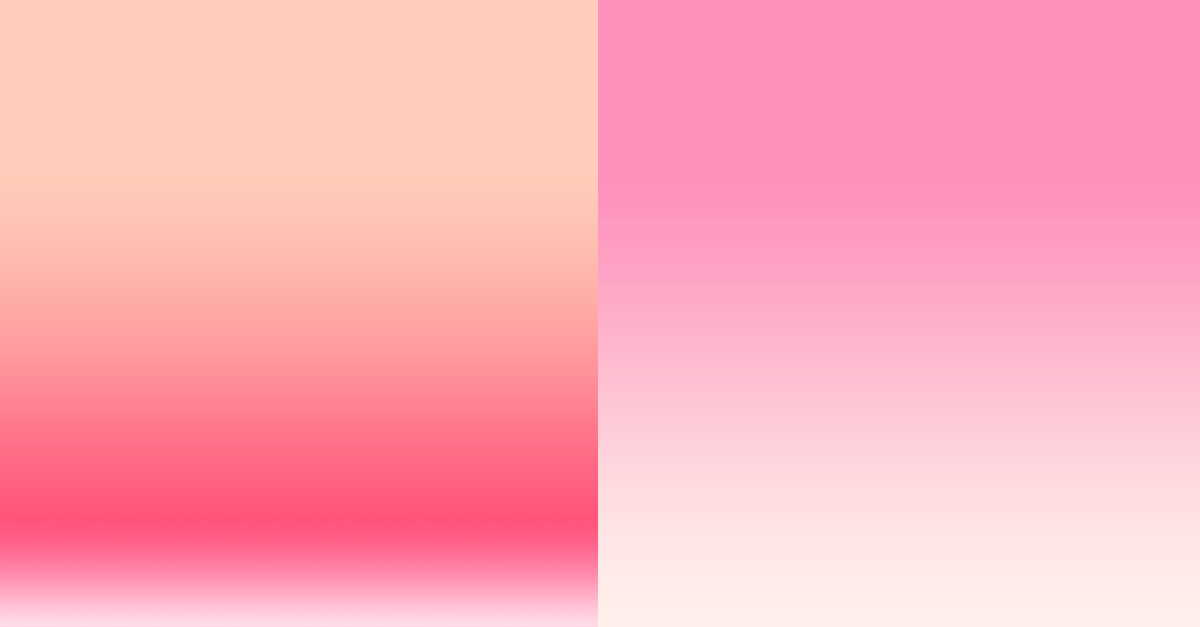
Multimodal generative models
Integrating various modalities into a unified generative model, generating and understanding joint modeling, supporting interleaved generation and simultaneous generation across various modalities (such as the digital avatar), and enhancing the contextual capabilities and consistency of generative models.
Multimodel
Diffusion Model
Auto Regression Model
Foundation
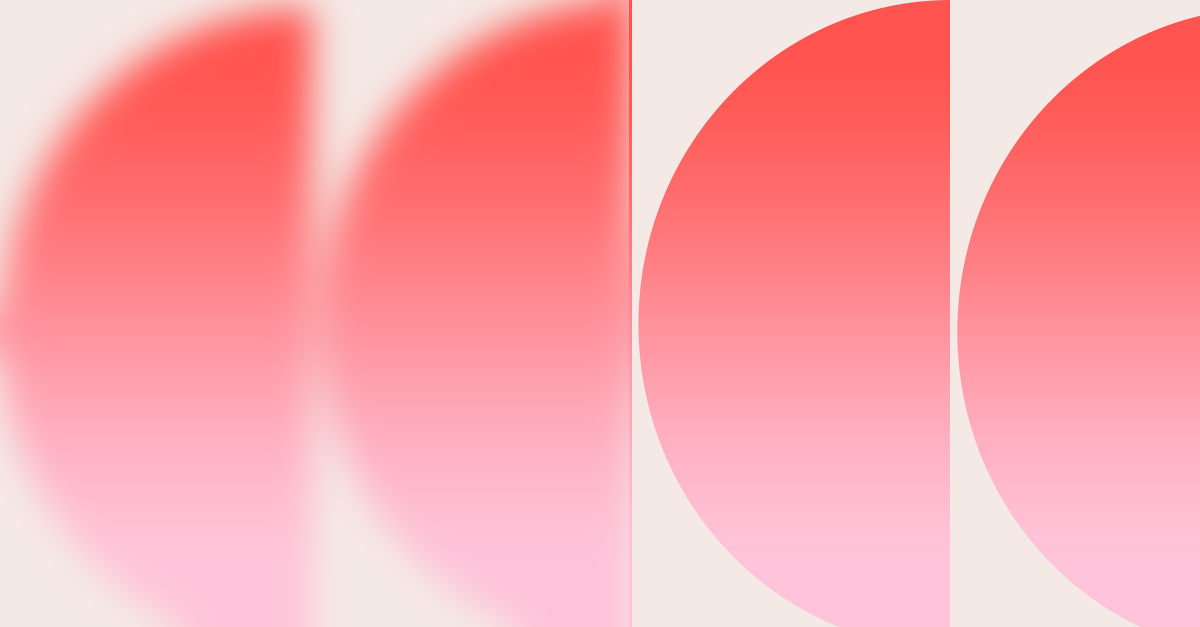
3D/4D generative models
3D/4D foundational generative models, learning visual world knowledge from video and 3D data, understanding the physical world's 3D space and physical laws, building spatial intelligence and world models, and exploring physics and rendering engines based on generative models.
3D
4D
World Model
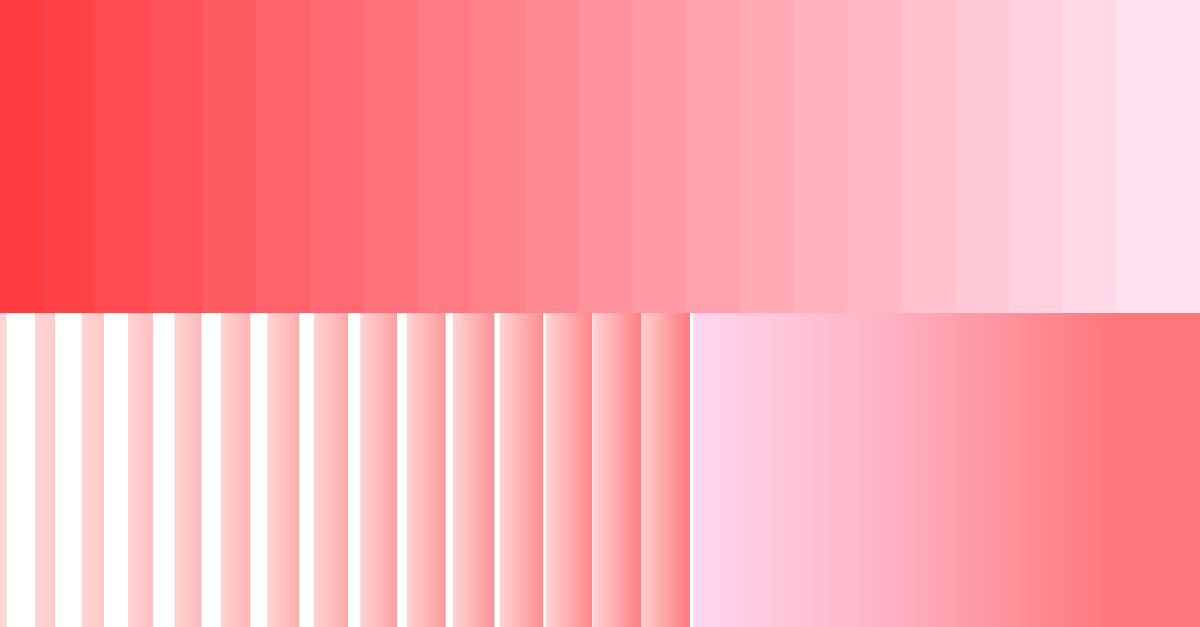
Multimodal model design and optimization
Design and optimize multimodal model network architectures, optimize diffusion models, carry out efficient large-scale distributed training and inference, and push for model acceleration and optimization.
Multimodal
Optimization
Distillation
Quantization
Selected Papers
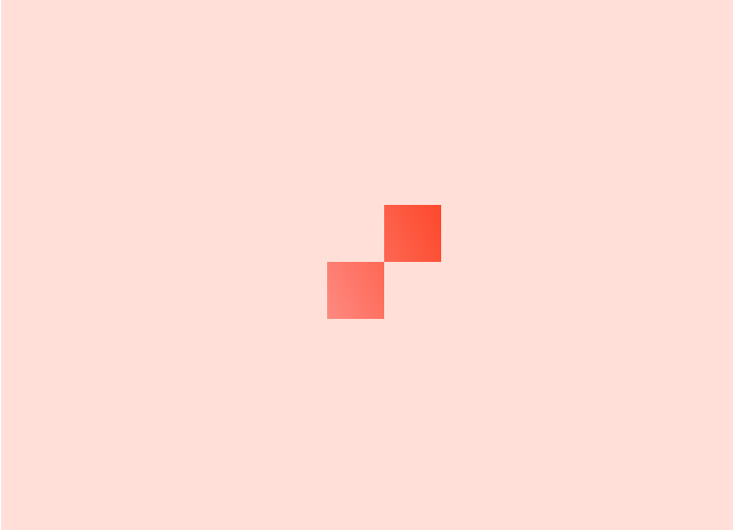
Apr 15, 2025
Seedream 3.0 Technical Report
We present Seedream 3.0, a high-performance Chinese-English bilingual image generation foundation model. We develop several technical improvements to address existing challenges in Seedream 2.0, including alignment with complicated prompts, fine-grained typography generation, suboptimal visual aesthetics and fidelity, and limited image resolutions. Specifically, the advancements of Seedream 3.0 stem from improvements across the entire pipeline, from data construction to model deployment. At the data stratum, we double the dataset using a defect-aware training paradigm and a dual-axis collaborative data-sampling framework. Furthermore, we adopt several effective techniques such as mixed-resolution training, cross-modality RoPE, representation alignment loss, and resolution-aware timestep sampling in the pre-training phase. During the post-training stage, we utilize diversified aesthetic captions in SFT, and a VLM-based reward model with scaling, thereby achieving outputs that well align with human preferences. Furthermore, Seedream 3.0 pioneers a novel acceleration paradigm. By employing consistent noise expectation and importance-aware timestep sampling, we achieve a 4 to 8 times speedup while maintaining image quality. Seedream 3.0 demonstrates significant improvements over Seedream 2.0: it enhances overall capabilities, in particular for text-rendering in complicated Chinese characters which is important to professional typography generation. In addition, it provides native high-resolution output (up to 2K), allowing it to generate images with high visual quality.
Seed Vision Team
Computer Vision
2025.04.15
Seedream 3.0 Technical Report
Seed Vision Team
Vision
Computer Vision
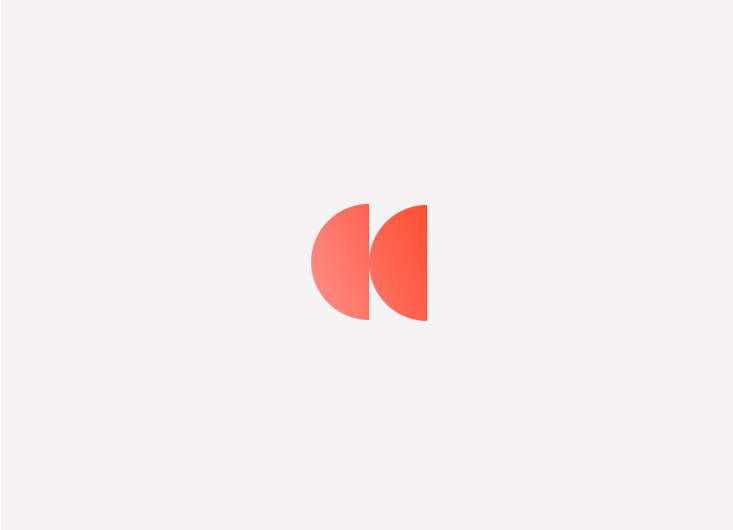
Mar 20, 2025
Multi-Reward as Condition for Instruction-based Image Editing
High-quality training triplets (instruction, original image, edited image) are essential for instruction-based image editing. Predominant training datasets (e.g., InsPix2Pix) are created using text-to-image generative models (e.g., Stable Diffusion, DALL-E) which are not trained for image editing. Accordingly, these datasets suffer from inaccurate instruction following, poor detail preserving, and generation artifacts. In this paper, we propose to address the training data quality issue with multi-perspective reward data instead of refining the ground-truth image quality. 1) we first design a quantitative metric system based on best-in-class LVLM (Large Vision Language Model), i.e., GPT-4o in our case, to evaluate the generation quality from 3 perspectives, namely, instruction following, detail preserving, and generation quality. For each perspective, we collected quantitative score in 0∼5 and text descriptive feedback on the specific failure points in ground-truth edited images, resulting in a high-quality editing reward dataset, i.e., RewardEdit20K. 2) We further proposed a novel training framework to seamlessly integrate the metric output, regarded as multi-reward, into editing models to learn from the imperfect training triplets. During training, the reward scores and text descriptions are encoded as embeddings and fed into both the latent space and the U-Net of the editing models as auxiliary conditions. 3) We also build a challenging evaluation benchmark with real-world images/photos and diverse editing instructions, named Real-Edit. Experiments indicate that our multi-reward conditioned model outperforms its no-reward counterpart on two popular editing pipelines, i.e., InsPix2Pix and SmartEdit. Code is released at this https URL[https://github.com/bytedance/Multi-Reward-Editing].
Xin Gu, Ming Li, Libo Zhang, Fan Chen, Longyin Wen, Tiejian Luo, Sijie Zhu
Computer Vision
2025.03.20
Multi-Reward as Condition for Instruction-based Image Editing
Xin Gu, Ming Li, Libo Zhang, Fan Chen, Longyin Wen, Tiejian Luo, Sijie Zhu
Vision
Computer Vision
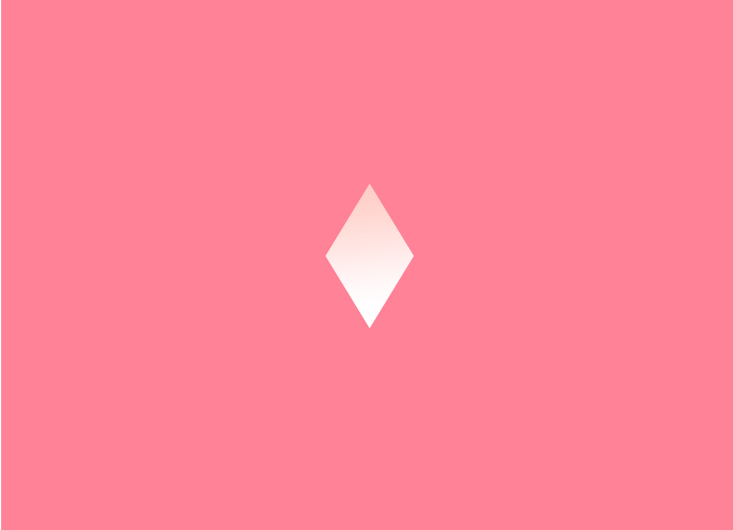
Mar 17, 2025
FlexWorld: Progressively Expanding 3D Scenes for Flexiable-View Synthesis
Generating flexible-view 3D scenes, including 360° rotation and zooming, from single images is challenging due to a lack of 3D data. To this end, we introduce FlexWorld, a novel framework consisting of two key components: (1) a strong video-to-video (V2V) diffusion model to generate high-quality novel view images from incomplete input rendered from a coarse scene, and (2) a progressive expansion process to construct a complete 3D scene. In particular, leveraging an advanced pre-trained video model and accurate depth-estimated training pairs, our V2V model can generate novel views under large camera pose variations. Building upon it, FlexWorld progressively generates new 3D content and integrates it into the global scene through geometry-aware scene fusion. Extensive experiments demonstrate the effectiveness of FlexWorld in generating high-quality novel view videos and flexible-view 3D scenes from single images, achieving superior visual quality under multiple popular metrics and datasets compared to existing state-of-the-art methods. Qualitatively, we highlight that FlexWorld can generate high-fidelity scenes with flexible views like 360° rotations and zooming.
Luxi Chen, Zihan Zhou, Min Zhao, Yikai Wang, Ge Zhang, Wenhao Huang, Hao Sun, Ji-Rong Wen, Chongxuan Li
Computer Vision
2025.03.17
FlexWorld: Progressively Expanding 3D Scenes for Flexiable-View Synthesis
Luxi Chen, Zihan Zhou, Min Zhao, Yikai Wang, Ge Zhang, Wenhao Huang, Hao Sun, Ji-Rong Wen, Chongxuan Li
Vision
Computer Vision
Learn More
Technical applications
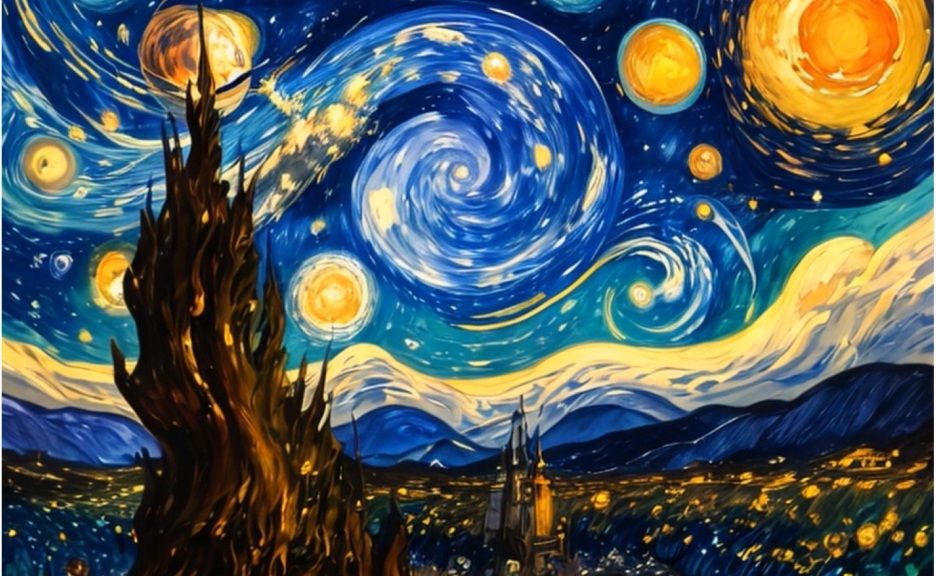
Doubao Text-to-Image
The Doubao Text-to-Image Model has been successfully integrated into products like Douyin, CapCut/Lark, Doubao, and StarSketch. Users can input prompts into the Doubao app to generate high-quality images that beautifully capture light and shadow, create rich color atmospheres, and depict character aesthetics. The model supports input in both Chinese and English, ensuring precise understanding of complex prompts.
Text-to-Image
Model
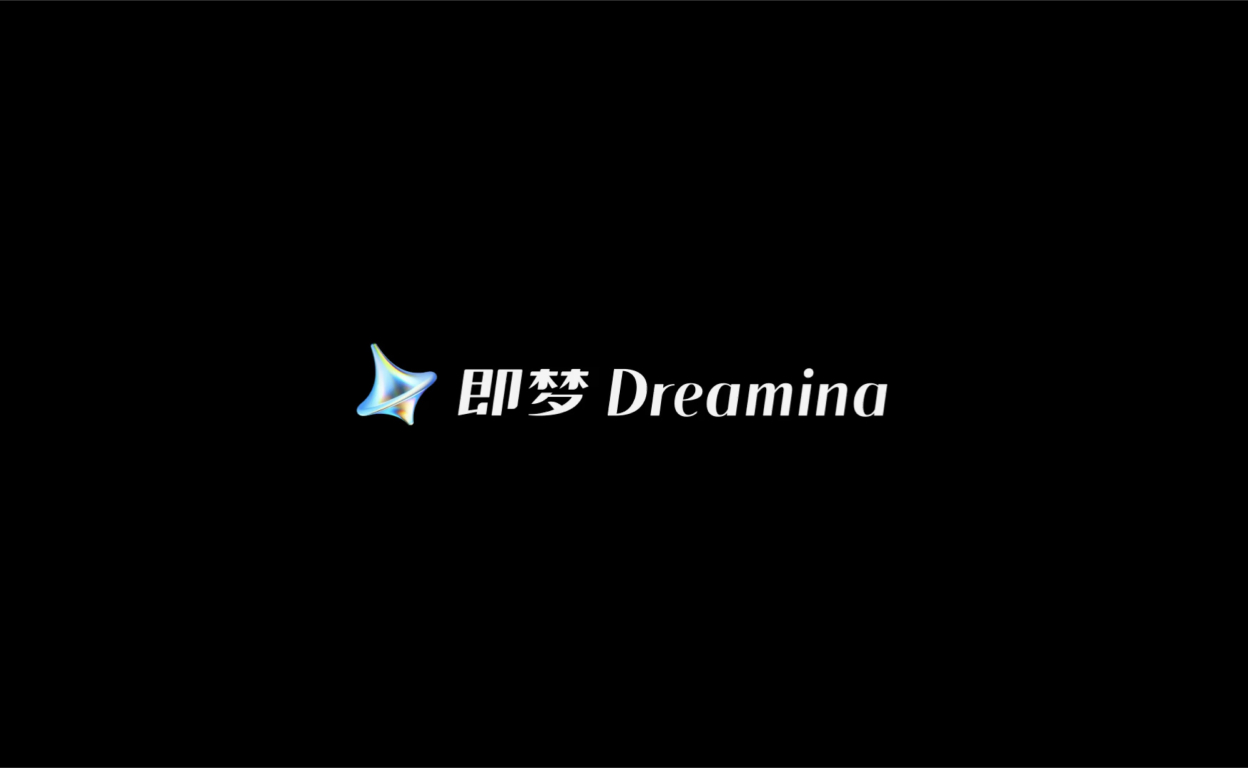
Jimeng
Jimeng/Dreamina is an AI-powered creative product developed by ByteDance. It enables users to generate high-quality images and videos through inputs in natural language and pictures. The platform provides an intelligent canvas, a story creation mode, and various AI editing tools, significantly boosting users' creative productivity.
AI-powered
Creative
Featured Jobs
Research Scientist, Multimodal Foundation Model
Research Scientist- Foundation Model, Video Generation
Research Engineer- Foundation Model AI Platform- San Jose
Research Scientist Graduate (Foundation Model, Video Generation) - 2025 Start (PhD)
Student Researcher (Doubao (Seed) - Foundation Model, Video Generation) - 2025 Start (PhD)
Student Researcher (Doubao (Seed) - Foundation Model AI Platform) - 2025 Start (PhD)